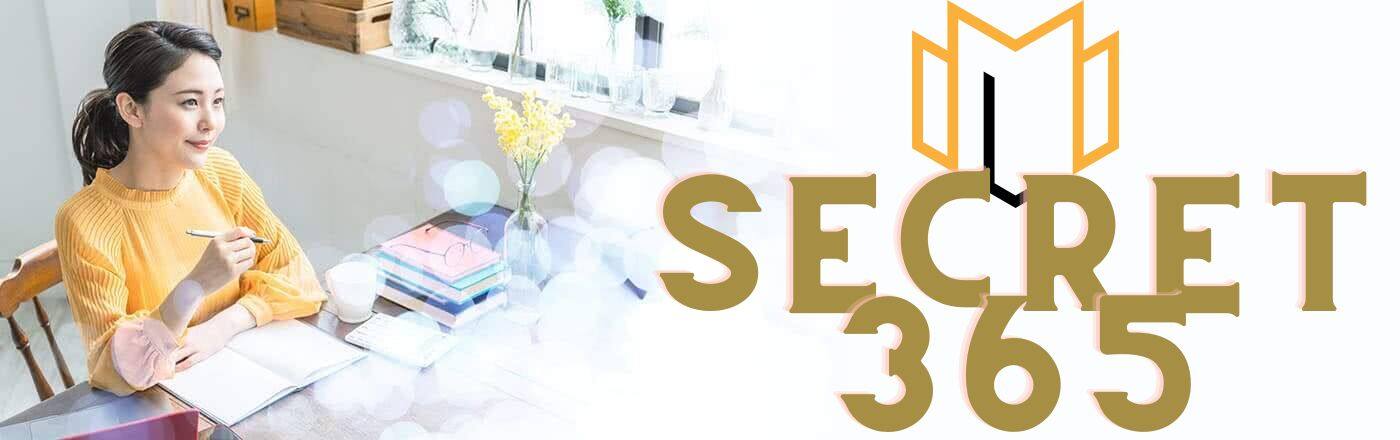
Unleashing the Future: Quantum Computing Revolutionizes AI
Quantum computing has emerged as a promising technology that has the potential to revolutionize various fields, including artificial intelligence (AI). While traditional computing systems have limitations in terms of processing power, quantum computing offers a new paradigm that can significantly enhance AI capabilities. This article explores the role of quantum computing in AI, going beyond processing power and delving into its potential to solve complex problems, improve machine learning algorithms, and enable breakthroughs in areas such as optimization, cryptography, and drug discovery.
Quantum computing has emerged as a promising technology that has the potential to revolutionize various fields, including artificial intelligence (AI). While much of the focus has been on the immense processing power that quantum computers can offer, their role in AI goes beyond just faster computations. In this article, we will explore how quantum computing is transforming AI algorithms and opening up new possibilities for solving complex problems.
One of the key advantages of quantum computing in AI lies in its ability to handle vast amounts of data and perform complex calculations simultaneously. Traditional computers, based on classical bits, process information in a binary format, either as a 0 or a 1. Quantum computers, on the other hand, use quantum bits or qubits, which can exist in multiple states simultaneously. This property, known as superposition, allows quantum computers to process and analyze multiple possibilities simultaneously, leading to exponential speedups in certain computations.
This increased processing power has significant implications for AI algorithms. Machine learning, a core component of AI, relies heavily on processing large datasets to identify patterns and make predictions. Quantum computers can accelerate this process by performing parallel computations, enabling faster training and more accurate models. This has the potential to revolutionize fields such as image recognition, natural language processing, and data analysis, where the sheer volume of data can be overwhelming for classical computers.
Another area where quantum computing is making a significant impact is in optimization problems. Many real-world problems, such as route optimization, portfolio management, and supply chain optimization, involve finding the best solution from a vast number of possibilities. Classical computers struggle with these problems due to the exponential growth in computation time as the number of possibilities increases. Quantum computers, with their ability to explore multiple possibilities simultaneously, can provide significant speedups in solving these optimization problems.
Quantum algorithms, such as the quantum approximate optimization algorithm (QAOA) and the quantum variational eigensolver (QVE), have been developed specifically to leverage the power of quantum computing in optimization. These algorithms have shown promising results in solving complex optimization problems, outperforming classical algorithms in terms of both speed and accuracy. As quantum computers continue to advance, we can expect further advancements in optimization algorithms, opening up new avenues for solving previously intractable problems.
While quantum computing holds great promise for AI, it is important to note that we are still in the early stages of its development. Quantum computers are highly sensitive to noise and errors, which can affect the accuracy of computations. Researchers are actively working on developing error-correcting codes and fault-tolerant architectures to mitigate these challenges. Additionally, the availability of practical quantum computers with a sufficient number of qubits is still limited, making it challenging to scale quantum algorithms to real-world problems.
Despite these challenges, the potential of quantum computing in AI is undeniable. As quantum computers continue to evolve and become more accessible, we can expect to see significant advancements in AI algorithms. From faster training of machine learning models to solving complex optimization problems, quantum computing has the potential to revolutionize the way we approach AI. It is an exciting time for researchers and practitioners in both quantum computing and AI, as they work together to unlock the full potential of this transformative technology.
Quantum computing has emerged as a promising technology that has the potential to revolutionize various fields, including artificial intelligence (AI). While much of the focus has been on the immense processing power that quantum computers offer, their role in AI goes beyond just faster computations. Quantum machine learning, in particular, has gained attention for its ability to enhance AI capabilities.
Traditional machine learning algorithms rely on classical computers to process and analyze vast amounts of data. However, as the complexity of AI tasks increases, classical computers face limitations in terms of computational power and efficiency. This is where quantum machine learning comes into play.
Quantum machine learning leverages the principles of quantum mechanics to perform computations in a fundamentally different way. Instead of using classical bits, which can represent either a 0 or a 1, quantum computers use quantum bits, or qubits, which can exist in a superposition of both 0 and 1 states simultaneously. This allows quantum computers to process and analyze multiple possibilities simultaneously, leading to exponential speedup in certain computations.
One of the key advantages of quantum machine learning is its ability to handle large-scale optimization problems. Optimization lies at the heart of many AI tasks, such as training neural networks or finding the best parameters for a given model. Classical optimization algorithms often struggle with the curse of dimensionality, where the number of possible solutions grows exponentially with the problem size. Quantum machine learning algorithms, on the other hand, can exploit quantum parallelism to search through a vast solution space more efficiently, potentially leading to faster and more accurate optimization.
Another area where quantum machine learning excels is in dealing with high-dimensional data. Traditional machine learning algorithms often struggle with high-dimensional feature spaces, as the number of parameters and computations required increases exponentially with the dimensionality. Quantum machine learning algorithms, however, can exploit quantum entanglement to capture complex correlations between features, even in high-dimensional spaces. This allows for more effective representation and analysis of data, leading to improved AI performance.
Furthermore, quantum machine learning offers the potential for enhanced privacy and security in AI applications. Quantum cryptography, for example, utilizes the principles of quantum mechanics to ensure secure communication and data encryption. By leveraging quantum properties such as entanglement and superposition, quantum machine learning algorithms can provide robust protection against cyber threats and unauthorized access to sensitive information.
Despite its immense potential, quantum machine learning is still in its early stages. The development of practical quantum algorithms and the availability of reliable quantum hardware remain significant challenges. However, researchers and industry experts are actively working towards overcoming these obstacles and exploring the possibilities of quantum machine learning in AI.
In conclusion, quantum machine learning holds great promise for enhancing AI capabilities beyond just processing power. By leveraging the principles of quantum mechanics, quantum machine learning algorithms can tackle large-scale optimization problems, handle high-dimensional data, and provide enhanced privacy and security. While there are still challenges to overcome, the potential impact of quantum machine learning on AI is undeniable. As the field continues to advance, we can expect to see exciting developments that push the boundaries of what AI can achieve.
Quantum computing has emerged as a promising technology that has the potential to revolutionize various fields, including artificial intelligence (AI). While much of the focus has been on the immense processing power that quantum computers can offer, their role in AI goes beyond just faster computations. Quantum neural networks, in particular, are advancing AI training and optimization, opening up new possibilities for solving complex problems.
Traditional neural networks, which are the backbone of AI systems, rely on classical computing to process and analyze data. However, as AI applications become more sophisticated and deal with increasingly complex datasets, the limitations of classical computing become apparent. This is where quantum neural networks come into play.
Quantum neural networks leverage the principles of quantum mechanics to enhance the capabilities of AI systems. Unlike classical neural networks, which use binary bits to represent information, quantum neural networks use quantum bits, or qubits, which can exist in multiple states simultaneously. This property, known as superposition, allows quantum neural networks to process and analyze vast amounts of data in parallel, leading to faster and more efficient computations.
One of the key advantages of quantum neural networks is their ability to handle exponentially large datasets. Traditional neural networks struggle with large datasets due to the computational complexity involved in processing and analyzing each data point. Quantum neural networks, on the other hand, can exploit the parallelism offered by qubits to process multiple data points simultaneously, significantly reducing the time and resources required for training and optimization.
Furthermore, quantum neural networks offer improved optimization capabilities. Optimization is a critical aspect of AI training, as it involves finding the best set of parameters that minimize the error between predicted and actual outputs. Classical optimization algorithms often get stuck in local minima, resulting in suboptimal solutions. Quantum neural networks, with their ability to explore multiple solutions simultaneously, can overcome this limitation and find global optima more efficiently.
Another area where quantum neural networks excel is in dealing with uncertainty and probabilistic reasoning. Traditional neural networks struggle with probabilistic tasks, as they rely on deterministic computations. Quantum neural networks, with their inherent probabilistic nature, can handle uncertain data and perform probabilistic reasoning more effectively. This opens up new possibilities for AI applications in fields such as finance, healthcare, and weather prediction, where uncertainty plays a significant role.
Despite the immense potential of quantum neural networks, there are still several challenges that need to be addressed. One of the major challenges is the issue of qubit stability and coherence. Qubits are highly sensitive to environmental noise and decoherence, which can lead to errors in computations. Researchers are actively working on developing error correction techniques and improving qubit stability to make quantum neural networks more reliable and practical.
In conclusion, quantum neural networks are advancing AI training and optimization by leveraging the power of quantum computing. With their ability to process large datasets in parallel, find global optima more efficiently, and handle uncertainty and probabilistic reasoning, quantum neural networks offer new avenues for solving complex problems. While there are challenges to overcome, the potential impact of quantum computing on AI is undeniable. As researchers continue to explore and develop this technology, we can expect to see significant advancements in AI capabilities in the near future.
1. What is the role of quantum computing in AI beyond processing power?
Quantum computing has the potential to enhance AI by enabling more efficient algorithms, improving machine learning capabilities, and solving complex optimization problems.
2. How does quantum computing enhance AI algorithms?
Quantum computing can improve AI algorithms by providing faster and more accurate data analysis, enabling better pattern recognition, and enhancing decision-making processes.
3. What complex problems can quantum computing help solve in AI?
Quantum computing can help solve complex optimization problems, such as resource allocation, route optimization, and portfolio optimization, which are challenging for classical computers to handle efficiently.
In conclusion, quantum computing has the potential to revolutionize the field of artificial intelligence by going beyond traditional processing power. Its ability to perform complex calculations and solve problems exponentially faster than classical computers opens up new possibilities for AI applications. Quantum computing can enhance machine learning algorithms, optimize data analysis, and improve decision-making processes. However, there are still significant challenges to overcome, such as error correction and scalability, before quantum computing can fully integrate into AI systems. Nonetheless, the role of quantum computing in AI holds great promise for advancing the capabilities and performance of AI technologies in the future.