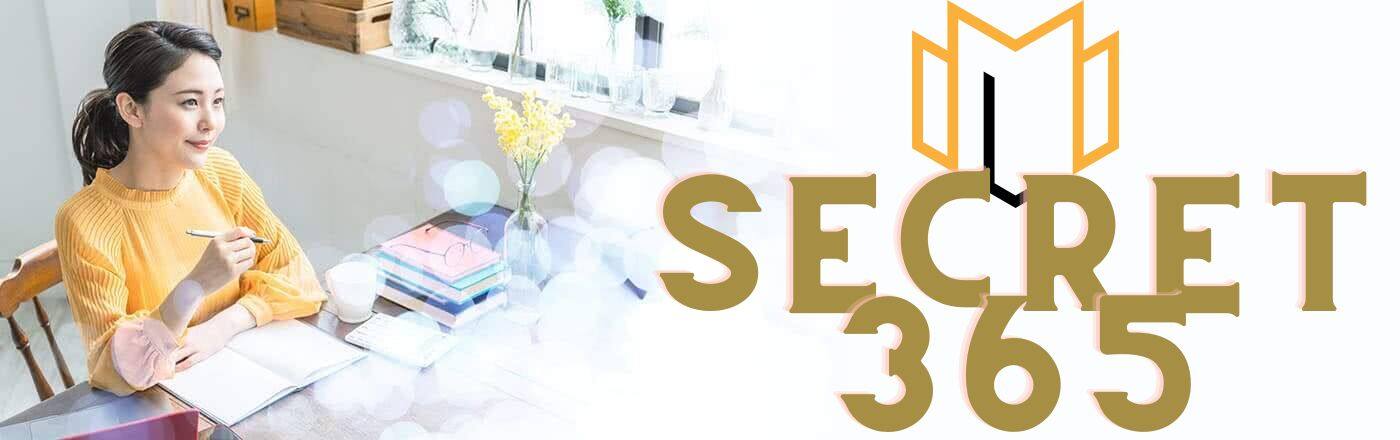
Unleash the Power of AI Retrieval with Advanced RAG Techniques in the Chroma Course.
This introduction is about exploring advanced RAG techniques for AI retrieval in the Chroma course.
Exploring Advanced RAG Techniques for AI Retrieval in the Chroma Course
Understanding the Role of RAG Techniques in AI Retrieval
Artificial Intelligence (AI) has become an integral part of our lives, revolutionizing various industries and enhancing our daily experiences. One of the key components of AI is information retrieval, which involves finding relevant information from a vast amount of data. To improve the efficiency and accuracy of AI retrieval, advanced techniques such as RAG (Retrieval-Augmented Generation) have been developed. In this article, we will explore the role of RAG techniques in AI retrieval, specifically in the context of the Chroma Course.
RAG techniques combine the power of retrieval models and language generation models to enhance the quality of AI retrieval. Traditionally, retrieval models focus on finding relevant documents based on a given query, while language generation models generate human-like responses. RAG techniques bridge the gap between these two approaches, allowing AI systems to not only retrieve relevant information but also generate coherent and contextually appropriate responses.
In the Chroma Course, RAG techniques play a crucial role in providing students with personalized and interactive learning experiences. By leveraging the power of AI retrieval, the Chroma Course can recommend relevant course materials, answer students' questions, and provide real-time feedback. This not only saves time for both students and instructors but also ensures that students receive tailored support throughout their learning journey.
One of the key advantages of RAG techniques is their ability to handle complex queries and generate informative responses. Traditional retrieval models often struggle with understanding the nuances of natural language queries, leading to inaccurate results. RAG techniques, on the other hand, can comprehend the context and intent behind a query, allowing for more accurate retrieval and generation of information. This is particularly beneficial in the Chroma Course, where students may have diverse learning needs and require specific information to progress effectively.
Furthermore, RAG techniques enable the Chroma Course to adapt and evolve based on user feedback. By continuously learning from user interactions, the AI system can improve its retrieval and generation capabilities over time. This iterative process ensures that the Chroma Course remains up-to-date and relevant, providing students with the most accurate and helpful information.
However, it is important to note that RAG techniques are not without challenges. One of the main concerns is the potential for biased or misleading information. As AI systems rely on pre-existing data to generate responses, there is a risk of perpetuating existing biases or misinformation. To mitigate this, the Chroma Course employs rigorous data filtering and validation processes, ensuring that the information provided is reliable and unbiased.
In conclusion, RAG techniques have revolutionized AI retrieval in the Chroma Course, enabling personalized and interactive learning experiences. By combining retrieval models with language generation models, RAG techniques enhance the accuracy and efficiency of information retrieval. They enable the Chroma Course to handle complex queries, generate informative responses, and adapt based on user feedback. While challenges such as bias and misinformation exist, the Chroma Course takes proactive measures to ensure the reliability and accuracy of the information provided. With the continued advancement of RAG techniques, the future of AI retrieval in education looks promising, offering students a truly immersive and tailored learning experience.
Exploring Advanced RAG Techniques for Improved AI Retrieval
Artificial Intelligence (AI) has become an integral part of our lives, revolutionizing various industries and enhancing our daily experiences. As AI continues to evolve, so does the need for efficient retrieval of information. One technique that has gained significant attention in recent years is the use of RAG (Retrieval-Augmented Generation) models. In this article, we will delve into the advanced RAG techniques used in the Chroma Course, shedding light on how they improve AI retrieval.
To understand the significance of RAG techniques, it is essential to grasp the concept of retrieval-augmented generation. Traditional AI models often struggle with generating accurate and contextually relevant responses. RAG models address this limitation by combining retrieval and generation approaches. They retrieve relevant information from a large knowledge base and then generate responses based on that retrieved information. This integration of retrieval and generation enables AI models to provide more accurate and comprehensive answers.
The Chroma Course, a leading platform for AI education, has embraced advanced RAG techniques to enhance the retrieval capabilities of its AI models. One such technique is the use of dense retrieval. Dense retrieval involves encoding the entire knowledge base into a dense vector space, allowing for efficient and accurate retrieval of information. By utilizing dense retrieval, the Chroma Course ensures that its AI models can quickly access relevant information, leading to improved retrieval performance.
Another advanced RAG technique employed by the Chroma Course is the use of passage ranking. Passage ranking involves ranking individual passages within a document based on their relevance to a given query. This technique allows the AI models to identify the most relevant passages, enabling them to generate more accurate and contextually appropriate responses. By incorporating passage ranking into their retrieval process, the Chroma Course ensures that its AI models can provide more precise and targeted information to users.
Furthermore, the Chroma Course leverages advanced RAG techniques such as negative sampling and hard negative mining. Negative sampling involves including negative examples during the training process to improve the model's ability to distinguish between relevant and irrelevant information. Hard negative mining, on the other hand, focuses on identifying challenging negative examples that are often misclassified by the model. By incorporating negative sampling and hard negative mining, the Chroma Course enhances the robustness and accuracy of its AI retrieval system.
Additionally, the Chroma Course utilizes a technique called query expansion to further improve AI retrieval. Query expansion involves expanding the original query with additional terms or synonyms to capture a broader range of relevant information. By expanding the query, the AI models can retrieve more comprehensive and diverse responses, enhancing the overall user experience. The Chroma Course's implementation of query expansion ensures that users receive a wider range of information, enabling them to explore various perspectives and insights.
In conclusion, advanced RAG techniques have significantly improved AI retrieval in the Chroma Course. By incorporating dense retrieval, passage ranking, negative sampling, hard negative mining, and query expansion, the Chroma Course ensures that its AI models can retrieve accurate, contextually relevant, and comprehensive information. These techniques not only enhance the retrieval capabilities of AI models but also contribute to a more enriching and satisfying user experience. As AI continues to advance, the exploration and implementation of advanced RAG techniques will undoubtedly play a crucial role in further improving AI retrieval systems.
Exploring Advanced RAG Techniques for AI Retrieval in the Chroma Course
Implementing RAG Techniques in the Chroma Course for Enhanced AI Retrieval
Artificial Intelligence (AI) has become an integral part of our lives, revolutionizing various industries and sectors. As AI continues to advance, the need for efficient retrieval of information becomes crucial. The Chroma Course, a leading online learning platform, recognizes this need and has implemented advanced RAG (Retrieval-Augmented Generation) techniques to enhance AI retrieval.
RAG techniques combine the power of both retrieval and generation models to provide more accurate and contextually relevant responses. By incorporating these techniques into the Chroma Course, students can benefit from a more personalized and interactive learning experience.
One of the key advantages of RAG techniques is their ability to understand the context of a query and generate responses accordingly. Traditional retrieval models often struggle with understanding the nuances of a question, leading to generic and sometimes irrelevant answers. With RAG techniques, the Chroma Course can now provide more precise and tailored responses, ensuring that students receive the information they need in a comprehensive manner.
Furthermore, RAG techniques enable the Chroma Course to go beyond simple text-based responses. By leveraging generation models, the platform can generate dynamic and interactive content, such as visualizations, simulations, and even virtual reality experiences. This not only enhances the learning experience but also allows students to engage with the material in a more immersive and hands-on way.
To implement RAG techniques effectively, the Chroma Course utilizes a combination of pre-training and fine-tuning. Pre-training involves training the model on a large corpus of text data, allowing it to learn the underlying patterns and structures of language. Fine-tuning, on the other hand, involves training the model on specific tasks and domains, such as the course material offered by the Chroma Course. This two-step process ensures that the model is both knowledgeable and contextually aware.
The Chroma Course also employs a continuous feedback loop to improve the performance of the RAG techniques. By collecting user feedback and monitoring the system's responses, the platform can identify areas for improvement and make necessary adjustments. This iterative process allows the Chroma Course to constantly enhance its AI retrieval capabilities, ensuring that students receive the most accurate and up-to-date information.
In addition to improving AI retrieval, RAG techniques also have the potential to transform the way assessments are conducted in the Chroma Course. Traditional multiple-choice questions can be limiting in assessing a student's understanding and critical thinking skills. With RAG techniques, the platform can generate more complex and open-ended questions, allowing students to demonstrate their knowledge in a more comprehensive manner. This not only provides a more accurate assessment of a student's abilities but also encourages deeper learning and engagement.
In conclusion, the Chroma Course has embraced advanced RAG techniques to enhance AI retrieval and provide a more personalized and interactive learning experience. By leveraging the power of retrieval and generation models, the platform can deliver contextually relevant responses and generate dynamic content. Through continuous feedback and improvement, the Chroma Course ensures that its AI retrieval capabilities are constantly evolving. With the implementation of RAG techniques, the Chroma Course is at the forefront of AI-driven education, empowering students to learn and grow in a more efficient and engaging manner.
1. What is the Chroma Course?
The Chroma Course is a program that focuses on exploring advanced RAG (Retrieval-Augmented Generation) techniques for AI retrieval.
2. What are RAG techniques?
RAG techniques refer to Retrieval-Augmented Generation techniques, which combine retrieval models with generative models to enhance AI retrieval capabilities.
3. What is the purpose of exploring advanced RAG techniques in the Chroma Course?
The purpose of exploring advanced RAG techniques in the Chroma Course is to improve AI retrieval capabilities by leveraging the power of retrieval models and generative models together.
In conclusion, exploring advanced RAG (Retrieval-Augmented Generation) techniques for AI retrieval in the Chroma course can greatly enhance the retrieval capabilities of AI systems. These techniques, such as using RAG models to generate relevant responses and incorporating retrieval-based methods for better context understanding, can improve the accuracy and relevance of information retrieval. By leveraging the power of RAG techniques, AI systems in the Chroma course can provide more comprehensive and accurate responses, leading to a more effective learning experience for users.