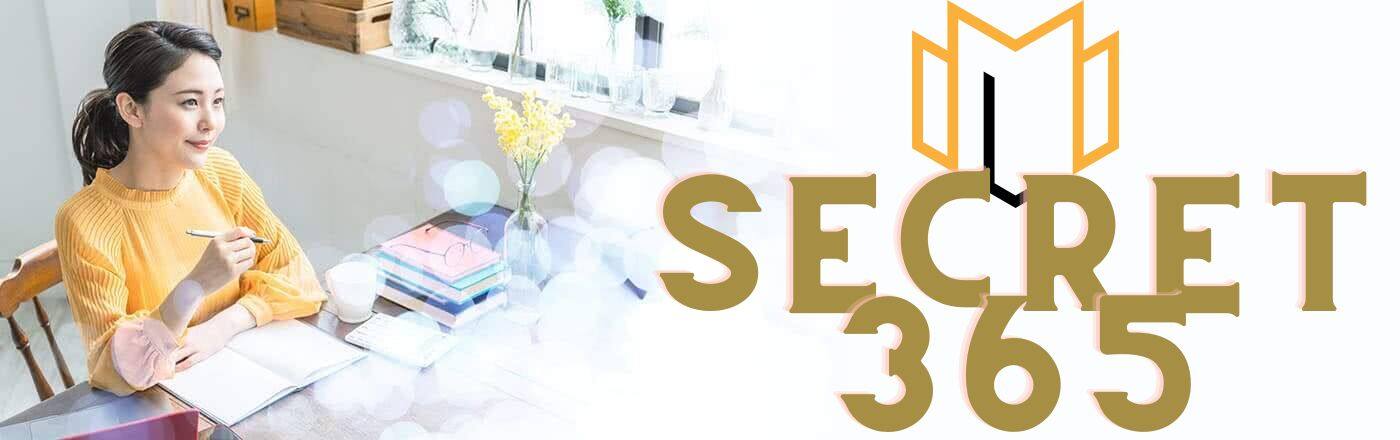
Exploring the Pros and Cons of Synthetic Data: Unveiling the Potential and Limitations.
Introduction:
Synthetic data refers to artificially generated data that mimics real-world data. It has gained significant attention in various fields, including machine learning, data analysis, and privacy protection. This article aims to explore the pros and cons of synthetic data, highlighting its potential benefits and drawbacks. By understanding the advantages and limitations of synthetic data, researchers and practitioners can make informed decisions regarding its usage in their respective domains.
Exploring the Pros and Cons of Synthetic Data
The Advantages of Synthetic Data in Machine Learning
Machine learning has become an integral part of various industries, from healthcare to finance, as organizations strive to leverage the power of data to make informed decisions. However, one of the biggest challenges in machine learning is the availability of high-quality, labeled data. This is where synthetic data comes into play, offering a potential solution to this problem.
Synthetic data refers to artificially generated data that mimics the statistical properties of real-world data. It is created using algorithms and models that replicate the patterns and characteristics of the original data. While synthetic data may not be derived from actual observations, it can still provide valuable insights and be used to train machine learning models. There are several advantages to using synthetic data in machine learning.
Firstly, synthetic data can address the issue of data scarcity. In many domains, obtaining large amounts of labeled data can be time-consuming, expensive, or even impossible due to privacy concerns. Synthetic data can be generated in abundance, allowing researchers and developers to create diverse datasets that cover a wide range of scenarios. This abundance of data enables more robust training of machine learning models, leading to better performance and generalization.
Secondly, synthetic data offers the advantage of data augmentation. Data augmentation is a technique used to artificially increase the size of a dataset by applying various transformations to the existing data. By augmenting the original data with synthetic samples, machine learning models can be exposed to a greater variety of instances, enhancing their ability to recognize patterns and make accurate predictions. This can be particularly useful in scenarios where the available real-world data is limited or lacks diversity.
Another advantage of synthetic data is its ability to address privacy concerns. In many applications, sensitive or personal information needs to be protected, making it challenging to share or use real-world data. Synthetic data can be generated in a way that preserves the statistical properties of the original data while removing any personally identifiable information. This allows organizations to share or publish synthetic datasets without compromising privacy, facilitating collaboration and research in sensitive domains.
Furthermore, synthetic data can be used to simulate rare or extreme events that are difficult to capture in real-world data. For example, in the field of autonomous driving, it is crucial to train models to handle rare and dangerous situations. By generating synthetic data that represents these scenarios, machine learning models can be trained to respond appropriately, improving their safety and reliability. Synthetic data enables researchers to explore the boundaries of their models and test their performance in challenging conditions.
Despite these advantages, it is important to acknowledge the limitations and potential drawbacks of synthetic data. One of the main concerns is the risk of introducing biases into the generated data. If the synthetic data does not accurately represent the underlying distribution of the real-world data, it can lead to biased models and inaccurate predictions. Careful validation and evaluation of the synthetic data generation process are necessary to ensure its effectiveness and reliability.
In conclusion, synthetic data offers several advantages in machine learning. It can address data scarcity, enable data augmentation, preserve privacy, and simulate rare events. However, it is crucial to carefully consider the limitations and potential biases associated with synthetic data. As machine learning continues to advance, synthetic data has the potential to play a significant role in overcoming the challenges of data availability and quality, ultimately leading to more accurate and robust models.
The use of synthetic data in data analysis has gained popularity in recent years. Synthetic data refers to artificially generated data that mimics the statistical properties of real data. While it offers several advantages, it is important to consider the disadvantages as well. In this section, we will explore the drawbacks of using synthetic data in data analysis.
One of the main disadvantages of synthetic data is the potential lack of accuracy. Since synthetic data is generated based on statistical models and assumptions, it may not fully capture the complexity and nuances of real-world data. This can lead to inaccurate results and misleading conclusions. For example, if the synthetic data fails to accurately represent the distribution of a certain variable, any analysis based on that variable may be flawed.
Another disadvantage of synthetic data is the potential for bias. Synthetic data is created based on existing data, and if the original data contains biases, those biases may be carried over to the synthetic data. This can perpetuate existing inequalities and discrimination. For instance, if the original data is biased towards a certain demographic group, the synthetic data may also exhibit the same bias, leading to biased analysis and decision-making.
Furthermore, the process of generating synthetic data can be time-consuming and resource-intensive. Creating synthetic data requires developing complex algorithms and models that accurately capture the statistical properties of the original data. This can be a time-consuming process, especially for large and complex datasets. Additionally, generating synthetic data may require significant computational resources, which can be costly and impractical for some organizations.
Another drawback of synthetic data is the potential for privacy concerns. Synthetic data is often created by perturbing or modifying real data to preserve privacy. However, there is always a risk that the synthetic data could still contain sensitive information that can be used to identify individuals or breach privacy. This is particularly concerning in industries that deal with highly sensitive data, such as healthcare or finance. Organizations must take appropriate measures to ensure that the synthetic data they generate and use does not compromise privacy.
Lastly, the lack of interpretability is a significant disadvantage of synthetic data. Since synthetic data is artificially generated, it may not have a clear and intuitive interpretation. This can make it challenging for analysts and decision-makers to understand and trust the results obtained from synthetic data analysis. Without a clear understanding of how the synthetic data was generated and what it represents, it becomes difficult to draw meaningful insights and make informed decisions based on the analysis.
In conclusion, while synthetic data offers several advantages in data analysis, it is important to consider its disadvantages as well. The potential lack of accuracy, bias, time and resource requirements, privacy concerns, and lack of interpretability are all factors that need to be carefully considered when using synthetic data. Organizations must weigh these drawbacks against the benefits and make informed decisions about whether or not to incorporate synthetic data into their data analysis processes.
Exploring the Ethical Implications of Using Synthetic Data
In recent years, the use of synthetic data has gained significant attention in various industries. Synthetic data refers to artificially generated data that mimics real-world data, but does not contain any personally identifiable information. While synthetic data offers several advantages, it also raises ethical concerns that need to be carefully considered.
One of the main advantages of using synthetic data is its ability to protect privacy. With the increasing amount of personal data being collected and stored, privacy has become a major concern. Synthetic data provides a solution by allowing organizations to analyze and share data without compromising individuals' privacy. By replacing sensitive information with synthetic data, organizations can ensure that no personal details are exposed, while still being able to perform meaningful analysis.
Another advantage of synthetic data is its potential to address data scarcity issues. In many industries, obtaining real-world data can be challenging due to various reasons such as legal restrictions or limited access. Synthetic data can be used to generate large volumes of data that closely resemble the real-world data, enabling organizations to overcome data scarcity challenges. This can be particularly beneficial in fields such as healthcare, where access to patient data is often restricted due to privacy concerns.
However, the use of synthetic data also raises ethical concerns that cannot be ignored. One of the main concerns is the potential for bias in the generated data. Synthetic data is created based on existing data, and if the original data contains biases, these biases can be replicated in the synthetic data. This can lead to biased analysis and decision-making, perpetuating existing inequalities and discrimination. It is crucial for organizations to carefully evaluate and address any biases present in the original data before generating synthetic data.
Another ethical concern is the potential for misuse of synthetic data. While synthetic data does not contain any personally identifiable information, it can still be used to infer sensitive information about individuals. For example, by analyzing patterns in synthetic data, it may be possible to identify individuals or uncover sensitive attributes. Organizations must ensure that appropriate safeguards are in place to prevent the misuse of synthetic data and protect individuals' privacy.
Additionally, the transparency and accountability of synthetic data generation methods are important ethical considerations. Organizations should be transparent about the methods used to generate synthetic data and ensure that these methods are reliable and accurate. It is also crucial to establish accountability mechanisms to address any issues or concerns that may arise from the use of synthetic data.
In conclusion, while synthetic data offers several advantages such as privacy protection and addressing data scarcity, it also raises ethical concerns that need to be carefully considered. The potential for bias, misuse, and lack of transparency in synthetic data generation methods must be addressed to ensure ethical use. Organizations must prioritize the ethical implications of using synthetic data and take appropriate measures to mitigate any potential risks. By doing so, they can harness the benefits of synthetic data while upholding ethical standards and protecting individuals' rights.
1. What are the pros of using synthetic data?
- Synthetic data can be generated quickly and in large quantities, saving time and resources.
- It allows for the creation of diverse and complex datasets that may be difficult or costly to obtain in real-world scenarios.
- Synthetic data can help protect privacy by removing personally identifiable information from the original dataset.
2. What are the cons of using synthetic data?
- Synthetic data may not accurately represent the real-world data it is intended to mimic, leading to potential biases or inaccuracies in analysis.
- It requires careful validation and testing to ensure that the synthetic data accurately reflects the characteristics and patterns of the original data.
- Synthetic data may not capture the full range of variability and complexity present in real-world data, limiting its usefulness in certain applications.
3. How can synthetic data be used?
- Synthetic data can be used for training machine learning models when real data is limited or unavailable.
- It can be used to augment existing datasets, increasing their size and diversity.
- Synthetic data can be used for testing and validation purposes, allowing for controlled experiments without the risk of exposing sensitive or private information.
In conclusion, exploring the pros and cons of synthetic data reveals several advantages and disadvantages. On the positive side, synthetic data offers a cost-effective and time-efficient solution for training machine learning models, as it eliminates the need for manual data collection. It also provides privacy protection by generating data that does not contain any personally identifiable information. Additionally, synthetic data allows for the creation of diverse and complex datasets that can simulate various scenarios and edge cases. However, synthetic data may lack the same level of accuracy and realism as real-world data, which can limit its effectiveness in certain applications. Furthermore, the process of generating synthetic data requires careful consideration and validation to ensure its quality and reliability. Overall, synthetic data presents both opportunities and challenges, and its suitability depends on the specific use case and requirements.