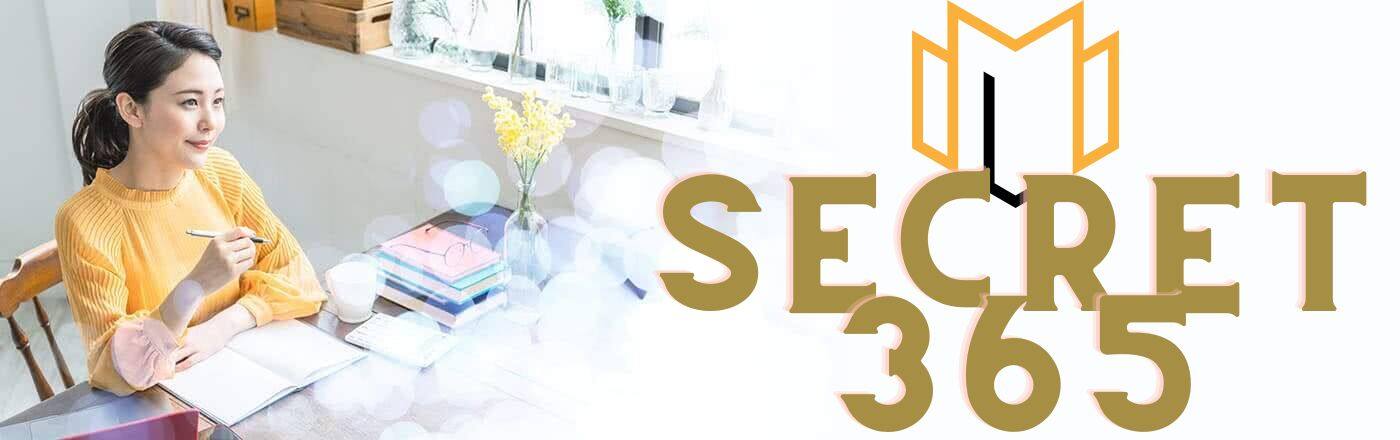
"Ensuring Language Models: Trustworthy, Safe, Fair, Robust, Private, and Beyond."
Evaluating language models for trustworthiness, safety, fairness, robustness, privacy, and more is crucial in ensuring the responsible and ethical deployment of AI systems. As language models become increasingly sophisticated and capable of generating human-like text, it is essential to assess their performance across various dimensions to mitigate potential risks and biases. This evaluation process helps identify and address any shortcomings or vulnerabilities, ensuring that language models meet the highest standards of reliability, security, fairness, and privacy. By thoroughly evaluating these models, we can foster trust in AI technologies and promote their responsible use in various domains.
Evaluating the Trustworthiness of Language Models: Methods and Challenges
Language models have become an integral part of our daily lives, powering virtual assistants, chatbots, and even generating news articles. As these models become more sophisticated, it is crucial to evaluate their trustworthiness. Trustworthiness encompasses various aspects, including safety, fairness, robustness, privacy, and more. In this article, we will delve into the methods and challenges of evaluating the trustworthiness of language models.
One of the primary concerns when evaluating language models is safety. Language models should not generate harmful or biased content that could potentially misinform or harm users. To assess safety, researchers employ techniques such as adversarial testing, where they intentionally input biased or harmful prompts to gauge the model's response. By analyzing the generated outputs, researchers can identify potential safety issues and work towards mitigating them.
Fairness is another critical aspect of trustworthiness. Language models should not exhibit biases based on factors such as race, gender, or religion. Evaluating fairness involves examining the model's responses to different demographic groups and identifying any disparities. Researchers employ fairness metrics and conduct bias analysis to ensure that the language model treats all users fairly and without discrimination.
Robustness is yet another crucial factor in evaluating the trustworthiness of language models. Robustness refers to a model's ability to handle inputs that deviate from the norm or contain errors. Robustness evaluation involves testing the model's performance on various challenging inputs, such as misspelled words, grammatically incorrect sentences, or ambiguous queries. By assessing the model's ability to handle such inputs, researchers can determine its robustness and identify areas for improvement.
Privacy is a growing concern in the era of language models. Users want assurance that their personal information remains secure and confidential. Evaluating privacy involves examining the model's data handling practices, including data storage, data anonymization, and data sharing policies. Researchers also assess the model's vulnerability to attacks that could compromise user privacy. By conducting privacy evaluations, developers can ensure that language models adhere to strict privacy standards.
However, evaluating the trustworthiness of language models is not without its challenges. One significant challenge is the lack of standardized evaluation methods. With the rapid advancement of language models, there is a need for consistent evaluation frameworks that encompass all aspects of trustworthiness. Researchers are actively working towards developing standardized evaluation metrics and benchmarks to facilitate fair comparisons between different models.
Another challenge lies in the dynamic nature of language models. Models are trained on vast amounts of data, which can include biased or harmful content. Even after rigorous evaluation, it is challenging to guarantee that a model will never generate biased or harmful outputs. Ongoing monitoring and continuous evaluation are necessary to address emerging issues and ensure that language models remain trustworthy over time.
Furthermore, evaluating trustworthiness requires interdisciplinary collaboration. Experts from various fields, including computer science, ethics, and social sciences, need to come together to develop comprehensive evaluation frameworks. This collaboration ensures that evaluations consider not only technical aspects but also ethical and societal implications.
In conclusion, evaluating the trustworthiness of language models is a complex task that involves assessing safety, fairness, robustness, privacy, and more. Researchers employ various methods, including adversarial testing, fairness metrics, and robustness evaluations, to evaluate these aspects. However, challenges such as the lack of standardized evaluation methods and the dynamic nature of language models persist. Interdisciplinary collaboration is crucial to developing comprehensive evaluation frameworks that address all aspects of trustworthiness. By continuously evaluating and improving language models, we can ensure that they remain reliable and trustworthy tools in our increasingly digital world.
Evaluating Language Models for Trustworthiness, Safety, Fairness, Robustness, Privacy, and More
Language models have become increasingly sophisticated and powerful, capable of generating human-like text that can fool even the most discerning reader. However, with great power comes great responsibility. As these models become more prevalent in our daily lives, it is crucial to evaluate them for various factors such as trustworthiness, safety, fairness, robustness, privacy, and more.
Ensuring the safety of language models is of paramount importance. These models have the potential to disseminate harmful or misleading information, leading to real-world consequences. To address this, researchers and developers have proposed several approaches. One such approach is pre-training models on a diverse range of data sources to minimize biases and improve fairness. By exposing the models to a wide array of perspectives, they can learn to generate more balanced and unbiased text.
Another consideration when evaluating language models is their robustness. Robustness refers to a model's ability to handle unexpected inputs or adversarial attacks. Adversarial attacks involve intentionally manipulating the input to trick the model into generating incorrect or harmful output. Evaluating a model's robustness involves testing it against various adversarial scenarios to ensure it can withstand such attacks.
Privacy is another crucial aspect to consider when evaluating language models. These models often require large amounts of data to train effectively, raising concerns about the privacy of individuals whose data is used. To address this, researchers have proposed techniques such as federated learning, where the training data remains on the user's device, and only the model updates are shared. This approach helps protect user privacy while still allowing the model to learn from a diverse range of data.
Trustworthiness is a fundamental factor when evaluating language models. Users need to have confidence in the information generated by these models. To ensure trustworthiness, models can be evaluated based on their ability to provide accurate and reliable information. This can be done by comparing the model's output to trusted sources or by incorporating human reviewers to validate the generated text.
Fairness is another critical consideration when evaluating language models. These models have the potential to perpetuate biases present in the training data, leading to unfair or discriminatory outputs. To address this, researchers have proposed techniques such as debiasing algorithms that aim to reduce biases in the model's output. Evaluating a model's fairness involves testing it against various sensitive attributes to ensure it does not discriminate or favor any particular group.
In addition to these factors, there are several other considerations when evaluating language models. One such consideration is interpretability, which refers to the ability to understand and explain the model's decision-making process. Interpretable models are crucial for ensuring transparency and accountability, especially in high-stakes applications such as healthcare or legal domains.
Another consideration is the model's adaptability to different domains or languages. Evaluating a model's adaptability involves testing its performance on various tasks or languages to ensure it can generalize well beyond its training data.
In conclusion, evaluating language models for trustworthiness, safety, fairness, robustness, privacy, and more is essential to ensure their responsible deployment in real-world applications. By considering these factors, researchers and developers can address potential risks and biases associated with these models, making them more reliable, fair, and secure. As language models continue to advance, it is crucial to prioritize their evaluation and improvement to build a future where AI-powered text generation benefits society as a whole.
Evaluating Language Models for Trustworthiness, Safety, Fairness, Robustness, Privacy, and More
Language models have become an integral part of our daily lives, powering virtual assistants, chatbots, and various other applications. As these models continue to evolve and become more sophisticated, it is crucial to assess their trustworthiness, safety, fairness, robustness, privacy, and other important factors. In this section, we will delve into the key considerations and techniques for assessing fairness, robustness, and privacy in language models.
Fairness is a critical aspect of language models, as they have the potential to perpetuate biases and discrimination. To evaluate fairness, it is essential to examine the training data used to develop the model. Bias in the training data can lead to biased outputs, reinforcing stereotypes or discriminating against certain groups. Techniques such as data augmentation, debiasing algorithms, and fairness metrics can help identify and mitigate biases in language models.
Robustness is another crucial factor to consider when evaluating language models. Robustness refers to the ability of a model to handle inputs that deviate from the norm or contain errors. Adversarial attacks, where inputs are intentionally manipulated to deceive the model, are a common concern. Evaluating robustness involves testing the model's performance under various conditions, including adversarial examples. Techniques like adversarial training and robustness metrics can help assess and improve the robustness of language models.
Privacy is a growing concern in the era of data-driven technologies. Language models often process sensitive information, raising concerns about data privacy. Evaluating privacy involves assessing the model's data handling practices, including data anonymization, encryption, and access controls. Privacy-preserving techniques such as differential privacy can be employed to protect user data while maintaining the model's utility.
In addition to fairness, robustness, and privacy, there are other important considerations when evaluating language models. Trustworthiness is paramount, as users need to have confidence in the model's outputs. Trust can be established through transparency, explainability, and accountability. Techniques like model interpretability and bias audits can help enhance trustworthiness.
Safety is another crucial aspect, especially in applications where language models interact with users. Models should be designed to prevent harmful or malicious behavior, such as generating inappropriate or offensive content. Safety evaluations involve testing the model's responses to various inputs, including those that could potentially trigger harmful behavior. Techniques like rule-based filtering and human-in-the-loop approaches can help ensure the safety of language models.
To assess these various factors, a combination of quantitative and qualitative evaluation methods is often employed. Quantitative metrics, such as accuracy, precision, and recall, provide objective measures of performance. Qualitative evaluations, such as user studies and expert reviews, offer insights into the model's real-world impact and user experience.
It is important to note that evaluating language models for fairness, robustness, privacy, and other factors is an ongoing process. As models continue to evolve and new challenges arise, evaluation techniques must adapt accordingly. Collaboration between researchers, developers, and policymakers is crucial to address these challenges and ensure the responsible development and deployment of language models.
In conclusion, assessing fairness, robustness, and privacy in language models is essential to mitigate biases, enhance performance, and protect user data. Evaluating these factors involves examining training data for biases, testing models under various conditions, and implementing privacy-preserving techniques. Trustworthiness and safety are also critical considerations. A combination of quantitative and qualitative evaluation methods is employed to assess these factors. As language models continue to advance, ongoing evaluation and collaboration are necessary to address emerging challenges and ensure responsible use.
1. What are some common methods for evaluating the trustworthiness of language models?
Some common methods for evaluating the trustworthiness of language models include conducting human evaluations to assess the accuracy and reliability of generated outputs, analyzing the model's performance on specific tasks or benchmarks, and examining the model's ability to handle adversarial inputs or detect misinformation.
2. How can the safety of language models be evaluated?
The safety of language models can be evaluated by assessing their potential to generate harmful or biased content. This can involve analyzing the model's outputs for offensive or inappropriate language, testing its ability to avoid generating false information, and examining its response to sensitive or harmful prompts.
3. What are some approaches to evaluating the fairness of language models?
Evaluating the fairness of language models involves assessing their potential biases towards certain demographic groups or underrepresented communities. Approaches to evaluating fairness can include analyzing the model's performance across different demographic groups, measuring the presence of biased language or stereotypes in generated outputs, and conducting user studies to assess the impact of the model's responses on different individuals.
In conclusion, evaluating language models for trustworthiness, safety, fairness, robustness, privacy, and more is crucial in ensuring their responsible deployment. It is essential to assess these models' performance in terms of generating accurate and reliable information, avoiding biased or harmful outputs, withstanding adversarial attacks, protecting user privacy, and addressing other ethical considerations. By conducting thorough evaluations, we can enhance the overall quality and reliability of language models, promoting their responsible and ethical use in various applications.