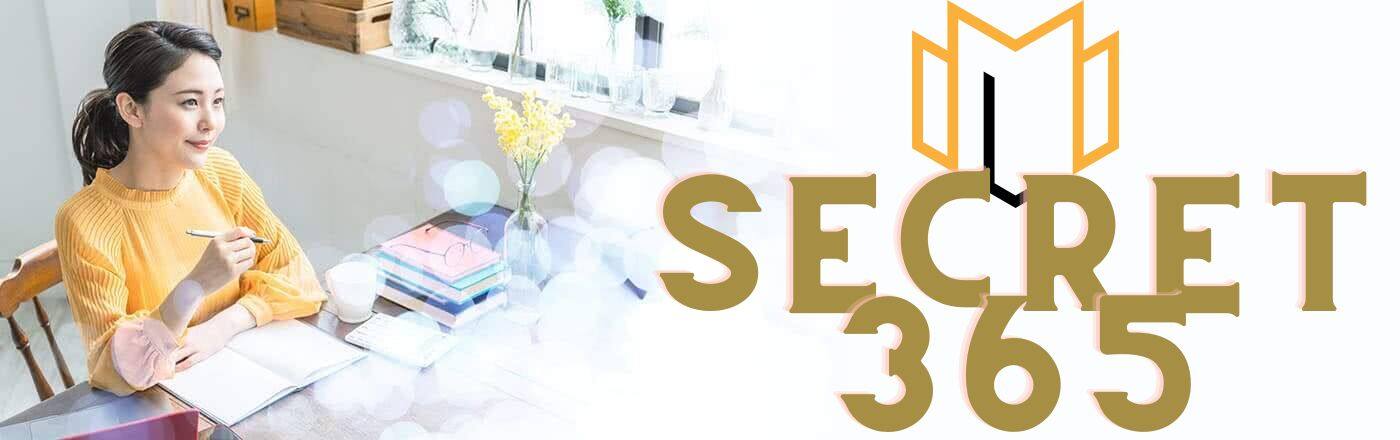
Accelerating Drug Development Through Machine Learning: The Role of AI in Drug Repurposing
Accelerating Drug Development Through Machine Learning: The Role of AI in Drug Repurposing
Machine learning and artificial intelligence (AI) have emerged as powerful tools in various fields, including drug development. In recent years, there has been a growing interest in utilizing AI to accelerate the process of drug repurposing. Drug repurposing involves finding new therapeutic uses for existing drugs, which can significantly reduce the time and cost associated with traditional drug discovery and development. This article explores the role of AI in drug repurposing and how it is revolutionizing the field of pharmaceutical research.
Accelerating Drug Development Through Machine Learning: The Role of AI in Drug Repurposing
Leveraging Machine Learning for Drug Repurposing: A Promising Approach
In the world of drug development, time is of the essence. The process of bringing a new drug to market is not only time-consuming but also incredibly expensive. However, recent advancements in artificial intelligence (AI) and machine learning have opened up new possibilities for accelerating drug development. One such area where AI is making a significant impact is in drug repurposing.
Drug repurposing, also known as drug repositioning, is the process of identifying new therapeutic uses for existing drugs. Traditionally, this has been a slow and labor-intensive process, relying on serendipitous discoveries or trial and error. However, with the advent of machine learning, researchers now have a powerful tool at their disposal to expedite this process.
Machine learning algorithms have the ability to analyze vast amounts of data and identify patterns that may not be immediately apparent to human researchers. By training these algorithms on large datasets of drug and disease information, researchers can uncover potential new uses for existing drugs. This approach has the potential to save both time and money in the drug development process.
One of the key advantages of using machine learning for drug repurposing is the ability to leverage existing knowledge. By analyzing data from clinical trials, electronic health records, and scientific literature, machine learning algorithms can identify potential connections between drugs and diseases. This allows researchers to focus their efforts on drugs that have already been approved for use in humans, bypassing the lengthy and costly process of developing new drugs from scratch.
Furthermore, machine learning algorithms can also help identify potential side effects or adverse reactions that may arise from repurposing a drug. By analyzing data on drug interactions and patient outcomes, these algorithms can provide valuable insights into the safety and efficacy of repurposed drugs. This information is crucial for regulatory agencies and pharmaceutical companies when making decisions about drug development.
Another advantage of using machine learning for drug repurposing is the ability to identify novel drug combinations. Many diseases are complex and multifactorial, requiring a combination of drugs to effectively treat them. Machine learning algorithms can analyze data on drug interactions and disease pathways to identify potential drug combinations that may have synergistic effects. This approach has the potential to revolutionize the way we treat diseases, offering new treatment options for patients.
Despite the promise of machine learning in drug repurposing, there are still challenges that need to be overcome. One of the main challenges is the availability and quality of data. Machine learning algorithms rely on large, diverse datasets to make accurate predictions. However, much of the data in the healthcare industry is fragmented and stored in different formats, making it difficult to access and analyze. Efforts are underway to address this issue, with initiatives such as data sharing platforms and standardized data formats.
In conclusion, machine learning has the potential to revolutionize drug repurposing and accelerate the drug development process. By leveraging existing knowledge and analyzing vast amounts of data, machine learning algorithms can identify new therapeutic uses for existing drugs, potentially saving time and money. Furthermore, machine learning can also help identify potential side effects and adverse reactions, as well as uncover novel drug combinations. While there are challenges to overcome, the future looks promising for the role of AI in drug repurposing.
Accelerating Drug Development Through Machine Learning: The Role of AI in Drug Repurposing
Enhancing Drug Development Efficiency with AI-powered Machine Learning
In recent years, the field of drug development has witnessed a remarkable transformation with the integration of artificial intelligence (AI) and machine learning (ML) techniques. These technologies have revolutionized the way drugs are discovered, developed, and repurposed. One area where AI-powered machine learning has shown great promise is in the field of drug repurposing, which aims to identify new therapeutic uses for existing drugs. By leveraging vast amounts of data and advanced algorithms, AI has the potential to significantly accelerate the drug development process, saving time, resources, and ultimately, lives.
Traditionally, drug development has been a slow and costly process, often taking years and billions of dollars to bring a new drug to market. However, with the advent of AI and ML, researchers now have access to powerful tools that can analyze vast amounts of data in a fraction of the time it would take a human. This has opened up new possibilities for drug repurposing, where existing drugs are screened for their potential to treat different diseases or conditions.
One of the key advantages of using AI in drug repurposing is its ability to analyze large datasets from various sources, including electronic health records, scientific literature, and clinical trial data. By integrating and analyzing this diverse range of information, AI algorithms can identify patterns and relationships that may not be apparent to human researchers. This can lead to the discovery of new therapeutic uses for existing drugs, potentially saving years of research and development.
Furthermore, AI-powered machine learning can also help predict the safety and efficacy of repurposed drugs. By training algorithms on historical data from clinical trials and adverse event reports, AI can identify potential risks and side effects associated with repurposed drugs. This can help researchers prioritize the most promising candidates for further investigation, reducing the time and cost of clinical trials.
Another area where AI is making a significant impact in drug repurposing is in the identification of drug combinations. Many diseases, such as cancer, require a combination of drugs to achieve optimal therapeutic effects. However, identifying the right combination of drugs can be a complex and time-consuming process. AI algorithms can analyze large datasets to identify potential drug combinations that may have synergistic effects, enhancing their therapeutic efficacy. This can lead to the development of more effective treatment regimens and improve patient outcomes.
Despite the tremendous potential of AI in drug repurposing, there are still challenges that need to be addressed. One of the main challenges is the availability and quality of data. AI algorithms rely on large, diverse datasets to make accurate predictions. However, much of the data in the healthcare industry is fragmented and stored in different formats, making it difficult to integrate and analyze. Efforts are underway to standardize and share data across different healthcare systems, which would greatly enhance the capabilities of AI in drug repurposing.
In conclusion, AI-powered machine learning has the potential to revolutionize drug development by accelerating the process of drug repurposing. By leveraging vast amounts of data and advanced algorithms, AI can identify new therapeutic uses for existing drugs, predict their safety and efficacy, and even identify synergistic drug combinations. While there are challenges to overcome, the integration of AI in drug repurposing holds great promise for improving the efficiency and effectiveness of drug development, ultimately benefiting patients worldwide.
Accelerating Drug Development Through Machine Learning: The Role of AI in Drug Repurposing
Revolutionizing Drug Discovery: The Impact of AI in Accelerating Drug Development
In recent years, the field of drug development has witnessed a remarkable transformation with the advent of artificial intelligence (AI) and machine learning. These technologies have revolutionized the way drugs are discovered, developed, and repurposed. One area where AI has shown immense potential is in drug repurposing, a process that involves finding new therapeutic uses for existing drugs. By leveraging the power of AI, researchers are now able to identify potential drug candidates for various diseases at an unprecedented speed and efficiency.
Traditionally, drug development has been a time-consuming and costly process. It typically takes several years and billions of dollars to bring a new drug to market. However, with the help of AI, this timeline can be significantly shortened. Machine learning algorithms can analyze vast amounts of data, including scientific literature, clinical trial results, and genomic data, to identify potential drug candidates. By sifting through this wealth of information, AI can quickly identify drugs that have the potential to be repurposed for different diseases.
One of the key advantages of using AI in drug repurposing is its ability to uncover hidden connections and patterns in data. AI algorithms can identify similarities between diseases based on their molecular profiles, allowing researchers to repurpose drugs that have already been approved for one condition to treat another. This approach not only saves time and resources but also increases the chances of success, as these repurposed drugs have already undergone rigorous testing for safety and efficacy.
Another way AI is accelerating drug development is through the prediction of drug-target interactions. By analyzing the molecular structure of drugs and the proteins they target, AI algorithms can predict how a drug will interact with its target and whether it is likely to be effective. This information is crucial in the early stages of drug development, as it helps researchers prioritize which drug candidates to pursue further. By using AI to predict drug-target interactions, researchers can focus their efforts on drugs that are more likely to succeed, saving valuable time and resources.
Furthermore, AI can also assist in the optimization of drug dosages and formulations. By analyzing patient data, including genetic information and clinical outcomes, AI algorithms can identify the optimal dosage and formulation for a particular drug. This personalized approach to drug development not only improves patient outcomes but also reduces the risk of adverse reactions and side effects.
Despite the numerous benefits of AI in drug repurposing, there are still challenges that need to be addressed. One of the main challenges is the availability and quality of data. AI algorithms rely on large, diverse datasets to make accurate predictions. However, much of the data in the field of drug development is proprietary and not easily accessible. Additionally, the quality of the data can vary, making it difficult for AI algorithms to make reliable predictions. To overcome these challenges, collaborations between pharmaceutical companies, academic institutions, and regulatory agencies are essential to ensure the availability and sharing of high-quality data.
In conclusion, AI has the potential to revolutionize drug development by accelerating the process of drug repurposing. By leveraging the power of machine learning, researchers can quickly identify potential drug candidates, predict drug-target interactions, and optimize dosages and formulations. While there are challenges to overcome, the benefits of AI in drug repurposing are undeniable. With continued advancements in AI technology and increased collaboration, we can expect to see even greater strides in drug development, ultimately leading to improved treatments and better patient outcomes.
1. How does machine learning accelerate drug development?
Machine learning algorithms can analyze large amounts of data, identify patterns, and make predictions, which can significantly speed up the drug development process by assisting in target identification, lead optimization, and toxicity prediction.
2. What is the role of AI in drug repurposing?
AI can play a crucial role in drug repurposing by analyzing existing drugs and their known properties, identifying potential new therapeutic uses, and predicting their efficacy in treating different diseases. This can save time and resources compared to developing new drugs from scratch.
3. What are the benefits of using AI in drug development?
Using AI in drug development can lead to faster and more efficient processes, reduced costs, and increased success rates in identifying potential drug candidates. It can also help uncover new therapeutic uses for existing drugs, leading to the repurposing of drugs for different diseases.
In conclusion, machine learning and AI have the potential to significantly accelerate drug development through drug repurposing. By leveraging large datasets and advanced algorithms, AI can identify new therapeutic uses for existing drugs, reducing the time and cost associated with traditional drug discovery processes. This approach holds promise for addressing urgent medical needs, improving patient outcomes, and driving innovation in the pharmaceutical industry.