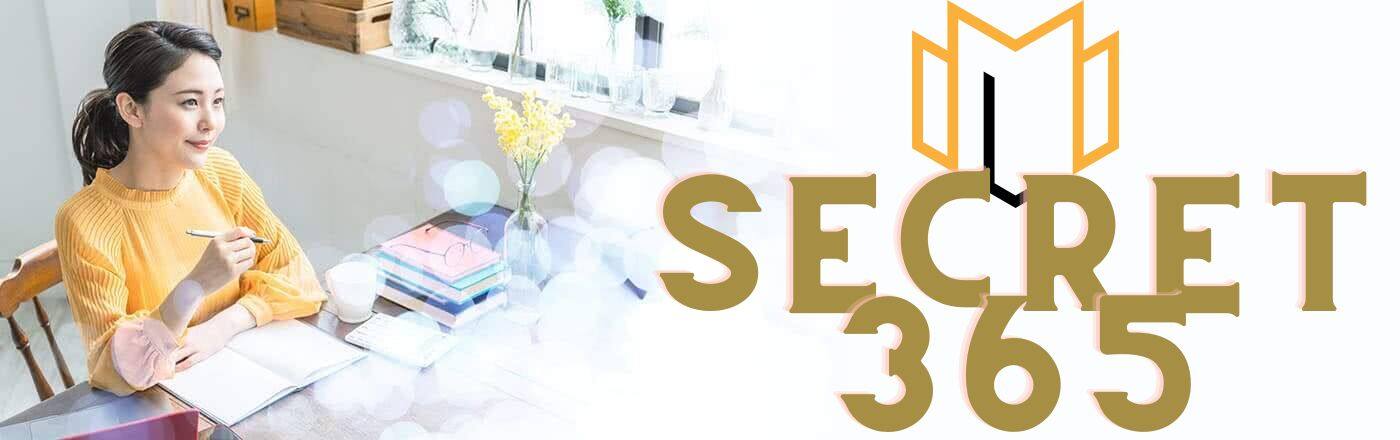
"Empowering Language Models with Expertise: Patent Practitioners Fueling AI's Knowledge Expansion."
The Role of Patent Practitioners in Generating Training Data for Large Language Models
Patent practitioners play a crucial role in generating training data for large language models. These models, such as OpenAI's GPT-3, require vast amounts of diverse and high-quality text data to learn and generate human-like responses. Patent practitioners possess the expertise and knowledge to create valuable training data by drafting patent applications, conducting prior art searches, and analyzing patent documents.
By drafting patent applications, practitioners generate a significant amount of technical and legal text that can be used to train language models. These applications contain detailed descriptions of inventions, including technical specifications, claims, and legal language. The diverse range of topics covered in patent applications helps in training language models to understand and generate accurate responses across various domains.
Additionally, patent practitioners conduct prior art searches to identify existing inventions and technologies relevant to a patent application. This process involves analyzing a vast amount of patent documents, scientific literature, and other sources of information. The expertise of patent practitioners in understanding complex technical concepts and legal terminology enables them to curate relevant and high-quality training data for language models.
Moreover, patent practitioners analyze patent documents to assess the novelty and inventiveness of an invention. This involves understanding the claims, descriptions, and legal aspects of patents. By leveraging their expertise, patent practitioners can contribute to the creation of training data that helps language models understand the nuances of patent language and generate accurate responses related to patent law and intellectual property.
In conclusion, patent practitioners play a vital role in generating training data for large language models. Their expertise in drafting patent applications, conducting prior art searches, and analyzing patent documents enables them to create diverse and high-quality text data. This data contributes to the training of language models, allowing them to generate human-like responses and assist in various domains, including patent law and intellectual property.
The field of artificial intelligence has seen significant advancements in recent years, particularly in the area of natural language processing. Large language models, such as OpenAI's GPT-3, have demonstrated impressive capabilities in understanding and generating human-like text. These models have the potential to revolutionize various industries, including healthcare, finance, and law. However, the success of these models heavily relies on the availability of high-quality training data. This is where patent practitioners play a crucial role.
Patent practitioners are professionals who specialize in the field of intellectual property law. They are responsible for drafting and prosecuting patent applications on behalf of inventors and companies. Their expertise lies in understanding complex technical concepts and translating them into a language that can be understood by patent examiners. This unique skill set makes them invaluable in generating training data for large language models.
One of the main challenges in training language models is the need for vast amounts of diverse and accurate data. These models learn from examples, and the more varied the examples, the better they become at understanding and generating text. Patent practitioners possess a wealth of technical documents, including patent applications, granted patents, and prior art references. These documents cover a wide range of topics, from cutting-edge technologies to obscure inventions. By leveraging this vast collection of documents, patent practitioners can contribute to the creation of diverse training data.
Furthermore, patent documents are written in a specific style and format that is distinct from other types of technical literature. They contain a combination of technical jargon, legal language, and descriptive text. This unique writing style presents a challenge for language models, as they need to be trained on data that closely resembles real-world documents. Patent practitioners can provide this valuable input by curating and annotating patent documents for training purposes.
In addition to providing training data, patent practitioners can also play a role in fine-tuning language models for specific applications. Large language models are trained on a vast corpus of text from the internet, which includes a wide range of topics and perspectives. However, certain domains, such as patent law, have their own specific terminology and nuances. By fine-tuning language models with domain-specific data provided by patent practitioners, these models can be customized to better understand and generate text in the context of patent law.
The involvement of patent practitioners in generating training data for large language models has several benefits. Firstly, it ensures that the models are trained on high-quality, accurate, and relevant data. This improves the overall performance and reliability of the models in real-world applications. Secondly, it allows patent practitioners to contribute to the advancement of artificial intelligence and its potential impact on the legal profession. By actively participating in the development of these models, patent practitioners can shape their capabilities to better serve their clients and the industry as a whole.
In conclusion, patent practitioners play a vital role in generating training data for large language models. Their expertise in understanding complex technical concepts and their access to a vast collection of patent documents make them invaluable in creating diverse and accurate training data. By leveraging their unique skill set, patent practitioners can contribute to the development of more advanced and specialized language models that have the potential to revolutionize the field of intellectual property law.
The development of large language models has revolutionized the field of natural language processing. These models, such as OpenAI's GPT-3, have the ability to generate human-like text and perform a wide range of language-related tasks. However, the training of these models requires massive amounts of data, and obtaining high-quality training data can be a challenging task. This is where patent practitioners play a crucial role.
Patent practitioners, also known as patent attorneys or agents, are professionals who specialize in intellectual property law. They work closely with inventors and companies to protect their inventions and innovations through the patenting process. In their day-to-day work, patent practitioners draft patent applications, conduct patent searches, and provide legal advice on patent-related matters. But their contributions go beyond just legal expertise.
One of the key responsibilities of patent practitioners is to draft patent applications that accurately describe the invention in detail. This involves not only understanding the technical aspects of the invention but also explaining it in a way that is clear and concise. This skill is invaluable when it comes to generating training data for large language models. The patent applications drafted by practitioners serve as a rich source of technical text that can be used to train these models.
The language used in patent applications is highly specialized and technical. It requires a deep understanding of the subject matter and the ability to communicate complex ideas effectively. Patent practitioners possess this expertise and are skilled at translating technical jargon into understandable language. This makes the text in patent applications ideal for training large language models, as it covers a wide range of technical domains and provides a diverse set of examples for the models to learn from.
Furthermore, patent applications are often written in a structured format, with sections dedicated to describing the background, summary, and detailed description of the invention. This structure provides a clear framework for organizing the training data and helps the models learn to generate text in a structured manner. Patent practitioners are well-versed in this format and can ensure that the patent applications they draft adhere to it, making the training data more consistent and reliable.
In addition to drafting patent applications, patent practitioners also conduct patent searches to assess the novelty and inventiveness of an invention. During this process, they review existing patents and technical literature to determine if the invention is truly unique. This involves analyzing and understanding a vast amount of technical text, which can be used to train large language models.
By leveraging their expertise in patent law and technical knowledge, patent practitioners contribute to the development of large language models in a unique way. They provide high-quality training data that is not only rich in technical content but also well-structured and consistent. This data helps improve the performance and capabilities of these models, enabling them to generate more accurate and contextually appropriate text.
In conclusion, patent practitioners play a crucial role in generating training data for large language models. Their expertise in drafting patent applications, conducting patent searches, and understanding technical text makes them valuable contributors to the development of these models. By leveraging their skills, patent practitioners help improve the accuracy and performance of large language models, paving the way for advancements in natural language processing.
Challenges Faced by Patent Practitioners in Generating Training Data for Large Language Models
Generating training data for large language models is a crucial task that requires careful consideration and expertise. Patent practitioners, who play a vital role in the patenting process, face unique challenges when it comes to generating training data for these models. In this article, we will explore some of the challenges faced by patent practitioners in this regard.
One of the primary challenges faced by patent practitioners is the sheer volume of patent documents that need to be processed. Patent databases contain millions of documents, each with its own unique language and technical jargon. This vast amount of data makes it difficult for patent practitioners to manually curate and annotate the training data required for large language models. The time and effort required to sift through these documents can be overwhelming, often leading to delays in generating the necessary training data.
Furthermore, patent documents are highly specialized and require domain-specific knowledge to understand and interpret. Patent practitioners need to have a deep understanding of various technical fields, such as engineering, biotechnology, or computer science, to accurately annotate the training data. This expertise is crucial in ensuring that the language models are trained on accurate and relevant data. However, acquiring and maintaining this level of expertise can be a challenge in itself, as it requires continuous learning and staying up-to-date with the latest advancements in multiple domains.
Another challenge faced by patent practitioners is the need for data privacy and confidentiality. Patent documents often contain sensitive information related to inventions and intellectual property. Maintaining the confidentiality of this information is of utmost importance. However, generating training data for large language models requires sharing and processing a significant amount of data. Patent practitioners must navigate this delicate balance between data privacy and the need for generating high-quality training data.
In addition to these challenges, patent practitioners also face the issue of data bias. Patent documents are written by inventors and their legal representatives, who may have their own biases and preferences. This bias can inadvertently be reflected in the training data, leading to biased language models. Patent practitioners need to be aware of this potential bias and take steps to mitigate it, such as ensuring diverse representation in the training data and using robust annotation guidelines.
To overcome these challenges, patent practitioners can leverage technology and automation. Natural language processing (NLP) tools can assist in the initial processing and filtering of patent documents, reducing the manual effort required. Machine learning algorithms can be used to identify relevant sections and extract key information from the documents, streamlining the data curation process. Additionally, collaboration platforms and secure data sharing mechanisms can be employed to ensure data privacy while facilitating the generation of training data.
In conclusion, patent practitioners face several challenges when it comes to generating training data for large language models. The volume and complexity of patent documents, the need for domain-specific expertise, data privacy concerns, and the potential for bias all contribute to the complexity of this task. However, by leveraging technology and adopting efficient processes, patent practitioners can overcome these challenges and contribute to the development of accurate and reliable language models that benefit various industries and domains.
1. What is the role of patent practitioners in generating training data for large language models?
Patent practitioners can contribute to generating training data for large language models by providing access to patent databases and assisting in the annotation and labeling of patent-related text.
2. How do patent practitioners assist in generating training data for large language models?
Patent practitioners can assist in generating training data by identifying relevant patent documents, extracting text data, and categorizing and labeling the data to create a comprehensive and diverse dataset for training large language models.
3. Why are patent practitioners important in generating training data for large language models?
Patent practitioners possess domain expertise and knowledge of patent-related language, making them valuable in generating accurate and specialized training data. Their involvement ensures that the language models are trained on high-quality patent-specific data, improving their performance in understanding and generating patent-related text.
In conclusion, patent practitioners play a crucial role in generating training data for large language models. Their expertise in understanding and analyzing patent documents allows them to curate and annotate relevant data that can be used to train these models. By providing accurate and comprehensive training data, patent practitioners contribute to the development of more advanced and effective language models, which can have significant implications in various fields such as natural language processing, information retrieval, and intellectual property research.