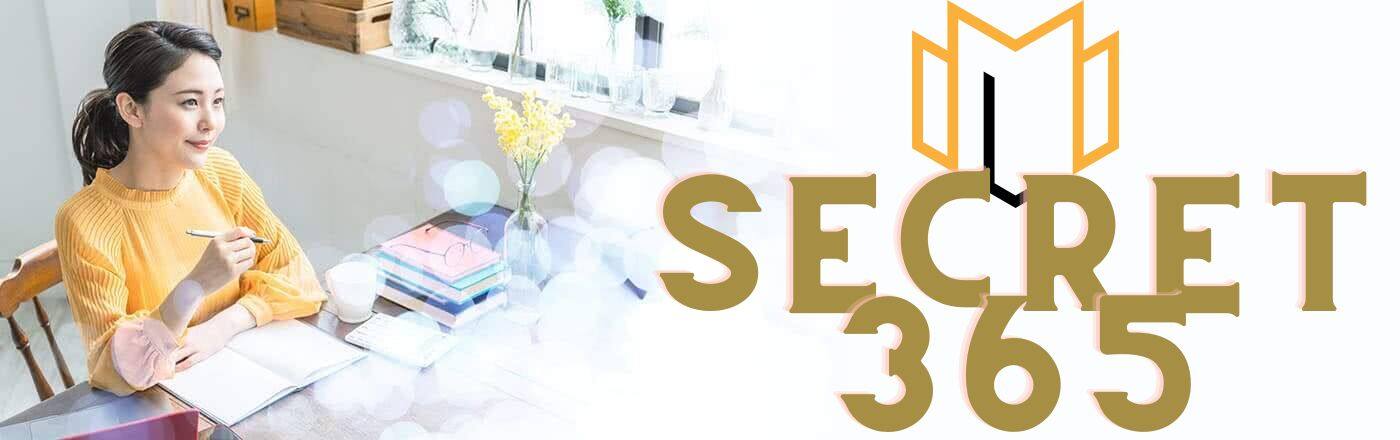
Unraveling the Genetic Code: Matrix Decomposition Revolutionizing Genomics.
Unraveling the Genetic Code: Matrix Decomposition's Groundbreaking Impact on Genomics
Matrix decomposition, a powerful mathematical technique, has made significant contributions to the field of genomics by aiding in the unraveling of the genetic code. Genomics, the study of an organism's complete set of DNA, has been revolutionized by the application of matrix decomposition methods. These methods have provided researchers with valuable insights into the complex relationships between genes, enabling a deeper understanding of genetic variations, disease mechanisms, and potential therapeutic targets. In this article, we will explore the groundbreaking impact of matrix decomposition on genomics and its role in advancing our knowledge of the genetic code.
Unraveling the Genetic Code: Matrix Decomposition's Groundbreaking Impact on Genomics
The field of genomics has made significant strides in recent years, thanks in large part to advancements in technology and computational methods. One such method that has revolutionized the field is matrix decomposition. Matrix decomposition, also known as matrix factorization, is a mathematical technique that breaks down a matrix into its constituent parts, allowing researchers to better understand the underlying structure and patterns within the data.
In the context of genomics, matrix decomposition has proven to be an invaluable tool for decoding the genetic code. The genetic code, which consists of the DNA sequences that make up an organism's genome, holds the key to understanding the fundamental building blocks of life. By deciphering this code, scientists can gain insights into the genetic basis of diseases, develop targeted therapies, and even explore the potential for genetic engineering.
Matrix decomposition techniques, such as singular value decomposition (SVD) and non-negative matrix factorization (NMF), have been particularly effective in uncovering the hidden patterns within genomic data. SVD, for example, breaks down a matrix into three separate matrices, each representing a different aspect of the data. This decomposition allows researchers to identify the most important features or genes that contribute to a particular phenotype or disease.
Similarly, NMF decomposes a matrix into two non-negative matrices, which can be interpreted as representing different biological processes or pathways. By applying NMF to genomic data, researchers can identify the underlying biological processes that are active in a particular cell type or tissue. This information can then be used to develop targeted therapies or interventions that specifically target these processes.
Matrix decomposition techniques have also been instrumental in identifying gene regulatory networks, which are the complex interactions between genes that control their expression. By decomposing gene expression data using techniques such as principal component analysis (PCA) or independent component analysis (ICA), researchers can identify the key regulators or drivers of gene expression. This knowledge can then be used to develop interventions that modulate these regulators, potentially leading to the development of new treatments for diseases.
Furthermore, matrix decomposition has proven to be a powerful tool for analyzing single-cell RNA sequencing data. Single-cell RNA sequencing allows researchers to measure gene expression in individual cells, providing unprecedented insights into cellular heterogeneity and dynamics. By decomposing the gene expression matrix using techniques such as non-negative matrix factorization or t-distributed stochastic neighbor embedding (t-SNE), researchers can identify distinct cell types or states within a population, as well as the genes that drive these differences.
In conclusion, matrix decomposition has had a groundbreaking impact on genomics by enabling researchers to unravel the genetic code. By breaking down complex genomic data into its constituent parts, matrix decomposition techniques have allowed researchers to identify hidden patterns, uncover gene regulatory networks, and analyze single-cell data. This newfound understanding of the genetic code has the potential to revolutionize medicine and lead to the development of targeted therapies for a wide range of diseases. As technology continues to advance, it is likely that matrix decomposition will play an even greater role in genomics, further unlocking the mysteries of the genetic code.
Unraveling the Genetic Code: Matrix Decomposition's Groundbreaking Impact on Genomics
Advancements in Genomics: Unraveling the Genetic Code through Matrix Decomposition
Genomics, the study of an organism's complete set of DNA, has revolutionized the field of biology. By understanding the genetic code, scientists can gain insights into the functioning of organisms, the development of diseases, and even the potential for personalized medicine. One of the key breakthroughs in genomics has been the use of matrix decomposition techniques to unravel the complex genetic code.
Matrix decomposition, also known as matrix factorization, is a mathematical technique that breaks down a matrix into its constituent parts. In the context of genomics, this technique is used to analyze large datasets of genetic information and identify patterns and relationships between genes. By decomposing the genetic matrix, scientists can uncover hidden structures and gain a deeper understanding of the genetic code.
One of the most widely used matrix decomposition techniques in genomics is singular value decomposition (SVD). SVD breaks down a matrix into three separate matrices: a left singular matrix, a diagonal matrix, and a right singular matrix. Each of these matrices contains valuable information about the genetic data.
The left singular matrix represents the relationship between genes, while the right singular matrix represents the relationship between samples or individuals. The diagonal matrix contains the singular values, which indicate the importance of each component in the decomposition. By analyzing these matrices, scientists can identify clusters of genes that are co-expressed or co-regulated, providing insights into the underlying biological processes.
Another powerful matrix decomposition technique used in genomics is non-negative matrix factorization (NMF). NMF is particularly useful when dealing with gene expression data, where each gene is associated with a numerical value representing its level of expression. By decomposing the gene expression matrix using NMF, scientists can identify groups of genes that are co-expressed under different conditions or in different tissues.
Matrix decomposition techniques have also been applied to other areas of genomics, such as genome-wide association studies (GWAS). GWAS involves analyzing the genetic variations across a population to identify genetic markers associated with diseases or traits. By decomposing the genetic matrix using techniques like principal component analysis (PCA), scientists can reduce the dimensionality of the data and identify the most important genetic variations.
The impact of matrix decomposition on genomics has been profound. It has allowed scientists to uncover complex relationships between genes, identify key biological processes, and even predict disease outcomes. By understanding the genetic code at a deeper level, researchers can develop targeted therapies and interventions that are tailored to an individual's genetic makeup.
However, there are still challenges to overcome in the field of genomics. The sheer volume of genetic data being generated is overwhelming, and the computational resources required for matrix decomposition can be substantial. Additionally, interpreting the results of matrix decomposition can be complex and requires expertise in both genomics and mathematics.
Despite these challenges, matrix decomposition continues to be a powerful tool in genomics research. As technology advances and computational resources become more accessible, scientists will be able to analyze larger and more complex datasets, further unraveling the mysteries of the genetic code.
In conclusion, matrix decomposition techniques have had a groundbreaking impact on genomics. By breaking down the genetic matrix into its constituent parts, scientists can uncover hidden structures and gain a deeper understanding of the genetic code. From identifying co-expressed genes to predicting disease outcomes, matrix decomposition has revolutionized the field of genomics and paved the way for personalized medicine. As we continue to unravel the genetic code, matrix decomposition will undoubtedly play a crucial role in advancing our understanding of biology and improving human health.
Unraveling the Genetic Code: Matrix Decomposition's Groundbreaking Impact on Genomics
Genomics, the study of an organism's complete set of DNA, has made significant strides in recent years. One of the key breakthroughs in this field has been the application of matrix decomposition techniques to understand the genetic code. Matrix decomposition, a mathematical method that breaks down complex data into simpler components, has revolutionized genomics by providing researchers with a powerful tool to analyze and interpret vast amounts of genetic information.
Matrix decomposition allows scientists to extract meaningful patterns and structures from large genomic datasets. By decomposing the data into its constituent parts, researchers can identify hidden relationships between genes, uncover gene regulatory networks, and gain insights into the underlying mechanisms of genetic diseases. This approach has proven particularly valuable in the field of personalized medicine, where understanding an individual's unique genetic makeup is crucial for tailoring treatments and interventions.
One of the most widely used matrix decomposition techniques in genomics is singular value decomposition (SVD). SVD breaks down a matrix into three separate matrices, each representing different aspects of the data. The first matrix captures the overall structure of the data, while the second and third matrices reveal the relationships between genes and samples. By analyzing these matrices, researchers can identify genes that are co-expressed or co-regulated, providing valuable information about their functional roles.
Another powerful matrix decomposition method used in genomics is non-negative matrix factorization (NMF). NMF decomposes a matrix into two non-negative matrices, which can be interpreted as representing different biological processes or cellular components. This technique has been instrumental in identifying gene expression signatures associated with specific diseases or biological processes. For example, NMF has been used to identify distinct subtypes of cancer based on gene expression patterns, leading to more targeted and effective treatments.
Matrix decomposition techniques have also been applied to analyze genomic sequence data. DNA sequences can be represented as matrices, with rows representing different sequences and columns representing the nucleotides at each position. By decomposing these matrices, researchers can identify conserved regions, discover sequence motifs, and predict the function of unknown genes. This approach has been particularly useful in studying the human genome, where understanding the function of non-coding regions has been a major challenge.
The impact of matrix decomposition on genomics extends beyond understanding the genetic code. It has also played a crucial role in deciphering the three-dimensional structure of the genome. Chromosome conformation capture techniques, such as Hi-C, generate large matrices that represent the interactions between different regions of the genome. By applying matrix decomposition methods to these matrices, researchers can identify topological domains, chromatin loops, and other structural features that are critical for gene regulation.
In conclusion, matrix decomposition has had a groundbreaking impact on genomics by providing researchers with a powerful tool to analyze and interpret complex genetic data. By breaking down large datasets into simpler components, matrix decomposition techniques have allowed scientists to uncover hidden relationships between genes, identify disease signatures, and understand the three-dimensional structure of the genome. As genomics continues to advance, matrix decomposition will undoubtedly play a central role in unraveling the mysteries of the genetic code and paving the way for personalized medicine.
1. What is matrix decomposition's impact on genomics?
Matrix decomposition has had a groundbreaking impact on genomics by providing a powerful tool for unraveling the genetic code.
2. How does matrix decomposition help in understanding the genetic code?
Matrix decomposition techniques allow researchers to analyze large genomic datasets and identify patterns and relationships within the genetic code. This helps in understanding the functions and interactions of genes and their role in various biological processes.
3. What are some specific applications of matrix decomposition in genomics?
Matrix decomposition has been used in genomics for tasks such as gene expression analysis, identifying gene regulatory networks, predicting protein-protein interactions, and studying genetic variations and mutations. These applications have greatly advanced our understanding of the genetic code and its implications in various diseases and biological phenomena.
In conclusion, matrix decomposition has had a groundbreaking impact on genomics by unraveling the genetic code. This mathematical technique has allowed researchers to analyze large-scale genomic data, identify patterns, and gain insights into the complex mechanisms underlying genetic information. Matrix decomposition methods have proven to be valuable tools in genomics research, enabling advancements in understanding genetic diseases, personalized medicine, and evolutionary biology. The application of matrix decomposition in genomics has opened up new avenues for studying and interpreting the vast amount of genetic data, ultimately leading to significant advancements in the field.