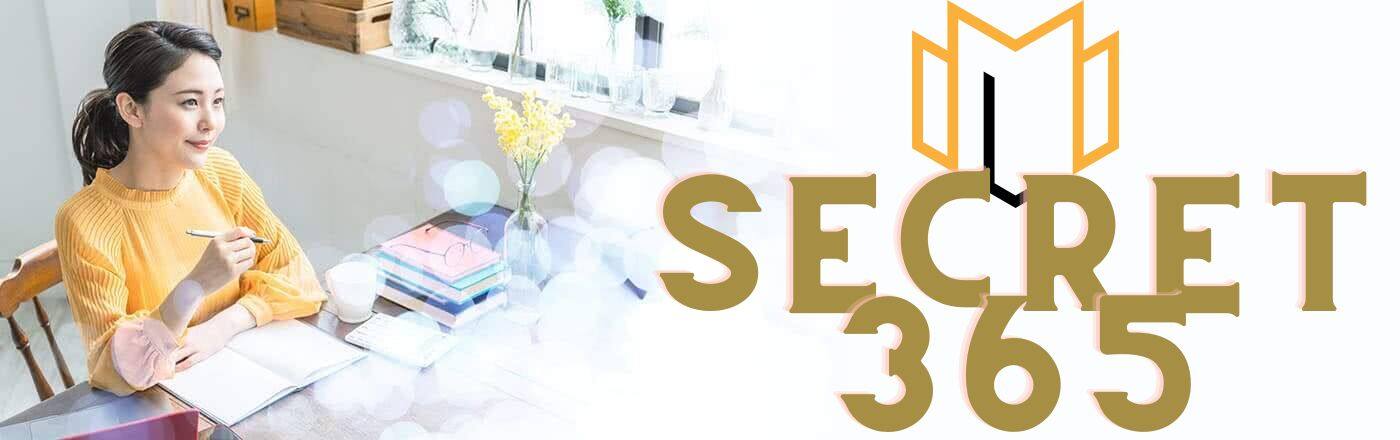
"Unlocking the Future of Lung Cancer Detection with Image Classification"
Introduction:
Predicting lung cancer through image classification is a significant area of research in the field of medical imaging. Lung cancer is one of the leading causes of cancer-related deaths worldwide, and early detection plays a crucial role in improving patient outcomes. Image classification techniques, combined with advanced machine learning algorithms, have shown promising results in accurately identifying lung cancer from medical images such as chest X-rays and CT scans. By analyzing these images, researchers aim to develop automated systems that can assist radiologists in detecting lung cancer at an early stage, enabling timely intervention and potentially saving lives.
The Role of Deep Learning in Predicting Lung Cancer through Image Classification
Lung cancer is a devastating disease that claims the lives of millions of people worldwide each year. Early detection is crucial for improving patient outcomes, as it allows for timely intervention and treatment. Traditional methods of diagnosing lung cancer involve invasive procedures such as biopsies, which can be uncomfortable for patients and may carry certain risks. However, recent advancements in deep learning and image classification have shown great promise in predicting lung cancer non-invasively.
Deep learning is a subset of machine learning that focuses on training artificial neural networks to learn and make predictions from large amounts of data. In the context of lung cancer prediction, deep learning algorithms can be trained on a vast number of medical images, such as chest X-rays and computed tomography (CT) scans. These algorithms learn to recognize patterns and features in the images that are indicative of lung cancer.
One of the key advantages of deep learning in predicting lung cancer is its ability to analyze images at a level of detail that is often beyond human capability. Deep learning algorithms can detect subtle changes in lung tissue that may be early signs of cancer, even before they are visible to the human eye. This level of sensitivity allows for the detection of lung cancer at its earliest stages, when it is most treatable.
To train deep learning algorithms for lung cancer prediction, large datasets of labeled medical images are required. These datasets consist of images that have been annotated by medical professionals to indicate the presence or absence of lung cancer. The deep learning algorithm is then trained on these labeled images, allowing it to learn the patterns and features associated with lung cancer.
Once the deep learning algorithm has been trained, it can be used to predict the presence or absence of lung cancer in new, unseen images. The algorithm analyzes the image and assigns a probability score indicating the likelihood of lung cancer. This score can then be used by medical professionals to guide further diagnostic tests or treatment decisions.
The accuracy of deep learning algorithms in predicting lung cancer has been shown to be comparable, and in some cases even superior, to that of human radiologists. A study published in the journal Nature Medicine found that a deep learning algorithm outperformed a team of radiologists in detecting lung cancer from CT scans. The algorithm achieved a 94.4% accuracy rate, compared to 65.5% for the radiologists.
While deep learning algorithms show great promise in predicting lung cancer, there are still challenges that need to be addressed. One of the main challenges is the need for large, high-quality datasets for training the algorithms. Collecting and annotating such datasets can be time-consuming and resource-intensive. Additionally, there is a need for standardized protocols and guidelines for training and evaluating deep learning algorithms in the context of lung cancer prediction.
In conclusion, deep learning and image classification have emerged as powerful tools in predicting lung cancer non-invasively. These algorithms have the potential to revolutionize lung cancer diagnosis by enabling early detection and intervention. However, further research and development are needed to overcome the challenges associated with training and evaluating deep learning algorithms. With continued advancements in this field, we can hope to improve patient outcomes and reduce the burden of lung cancer worldwide.
Advancements in Medical Imaging Techniques for Predicting Lung Cancer
Medical imaging has revolutionized the field of healthcare, enabling doctors to diagnose and treat diseases with greater accuracy. One area where medical imaging has made significant strides is in the prediction of lung cancer. Through the use of image classification techniques, doctors can now identify potential cancerous growths in the lungs at an early stage, increasing the chances of successful treatment and improved patient outcomes.
One of the most commonly used imaging techniques for predicting lung cancer is computed tomography (CT) scanning. CT scans provide detailed cross-sectional images of the lungs, allowing doctors to detect abnormalities that may indicate the presence of cancer. These images are then analyzed using image classification algorithms, which can differentiate between normal lung tissue and cancerous lesions.
Machine learning algorithms play a crucial role in image classification for lung cancer prediction. These algorithms are trained on large datasets of CT scans, with each scan labeled as either cancerous or non-cancerous. By analyzing the features and patterns present in these labeled scans, the algorithms learn to identify the characteristics of lung cancer. Once trained, these algorithms can accurately classify new CT scans, providing doctors with a valuable tool for early detection.
One of the key challenges in lung cancer prediction through image classification is the presence of false positives and false negatives. False positives occur when the algorithm incorrectly identifies a non-cancerous lesion as cancerous, leading to unnecessary follow-up tests and anxiety for the patient. On the other hand, false negatives occur when the algorithm fails to identify a cancerous lesion, potentially delaying diagnosis and treatment. Researchers are constantly working to improve the accuracy of image classification algorithms to minimize these errors.
To address the challenge of false positives and false negatives, researchers are exploring the use of deep learning techniques. Deep learning algorithms, inspired by the structure of the human brain, can analyze complex patterns and features in medical images. These algorithms have shown promising results in reducing false positives and false negatives in lung cancer prediction. By leveraging the power of deep learning, doctors can have more confidence in the accuracy of image classification for lung cancer.
Another area of advancement in medical imaging for lung cancer prediction is the integration of multiple imaging modalities. While CT scans are the most commonly used imaging technique, other modalities such as positron emission tomography (PET) and magnetic resonance imaging (MRI) can provide complementary information. By combining the data from multiple imaging modalities, doctors can improve the accuracy of lung cancer prediction and reduce the chances of false positives and false negatives.
In conclusion, advancements in medical imaging techniques have greatly improved the prediction of lung cancer. Through the use of image classification algorithms, doctors can now identify potential cancerous growths in the lungs at an early stage, increasing the chances of successful treatment. However, challenges such as false positives and false negatives still exist, and researchers are actively working to improve the accuracy of image classification algorithms. The integration of deep learning techniques and the combination of multiple imaging modalities show promise in further enhancing the accuracy of lung cancer prediction. With continued research and development, medical imaging will continue to play a vital role in the early detection and treatment of lung cancer.
Predicting Lung Cancer through Image Classification: Challenges and Future Directions
Lung cancer is one of the leading causes of cancer-related deaths worldwide. Early detection plays a crucial role in improving patient outcomes and survival rates. In recent years, image classification techniques have emerged as a promising tool for predicting lung cancer. By analyzing medical images, such as computed tomography (CT) scans, researchers can identify patterns and abnormalities that may indicate the presence of cancer. However, despite the potential benefits, there are several challenges that need to be addressed to further enhance the accuracy and reliability of lung cancer prediction through image classification.
One of the primary challenges in predicting lung cancer through image classification is the availability of high-quality and diverse datasets. Machine learning algorithms heavily rely on large and diverse datasets to learn and generalize patterns effectively. However, obtaining such datasets can be challenging due to privacy concerns and the limited availability of annotated medical images. To overcome this challenge, collaborations between healthcare institutions and research organizations are crucial. By pooling resources and sharing data, researchers can create comprehensive datasets that capture the full spectrum of lung cancer cases, enabling more accurate predictions.
Another challenge lies in the interpretation and standardization of medical images. Radiologists play a vital role in diagnosing lung cancer, but their interpretations can be subjective and prone to human error. Moreover, different imaging techniques and protocols can lead to variations in image quality and presentation. These factors can significantly impact the performance of image classification algorithms. To address this challenge, efforts should be made to develop standardized imaging protocols and establish guidelines for radiologists to ensure consistent and reliable interpretations. Additionally, the integration of artificial intelligence (AI) algorithms into radiology workflows can assist radiologists in making more accurate and efficient diagnoses.
Furthermore, the complexity and heterogeneity of lung cancer pose significant challenges for image classification. Lung cancer can manifest in various forms, including different histological subtypes and stages. Additionally, the presence of comorbidities and other lung diseases can further complicate the classification process. To overcome this challenge, researchers are exploring the use of deep learning techniques, such as convolutional neural networks (CNNs), which have shown promising results in image classification tasks. These advanced algorithms can automatically learn hierarchical representations from medical images, enabling them to capture subtle patterns and variations associated with different types of lung cancer.
Looking ahead, there are several future directions that hold promise for improving lung cancer prediction through image classification. Firstly, the integration of multi-modal imaging data, such as combining CT scans with positron emission tomography (PET) or magnetic resonance imaging (MRI), can provide a more comprehensive view of lung cancer and enhance the accuracy of predictions. Secondly, the development of explainable AI models can help address the black-box nature of deep learning algorithms, enabling clinicians to understand and trust the predictions made by these models. Lastly, the application of transfer learning, where pre-trained models are fine-tuned on specific lung cancer datasets, can help overcome the limitations of limited training data and improve the generalization capabilities of image classification algorithms.
In conclusion, predicting lung cancer through image classification holds great potential for improving early detection and patient outcomes. However, several challenges need to be addressed to enhance the accuracy and reliability of these predictions. By addressing issues related to data availability, interpretation and standardization of medical images, and the complexity of lung cancer, researchers can pave the way for more effective image classification algorithms. Additionally, future directions such as the integration of multi-modal imaging data, explainable AI models, and transfer learning offer exciting opportunities for further advancements in this field. With continued research and collaboration, image classification can become an invaluable tool in the fight against lung cancer.
1. How can image classification be used to predict lung cancer?
Image classification can be used to predict lung cancer by analyzing medical images, such as chest X-rays or CT scans, and identifying patterns or abnormalities that are indicative of lung cancer.
2. What are the benefits of using image classification for predicting lung cancer?
Using image classification for predicting lung cancer offers several benefits, including early detection of lung cancer, which can lead to better treatment outcomes. It can also help in reducing the need for invasive procedures and unnecessary biopsies.
3. What challenges are associated with predicting lung cancer through image classification?
Some challenges associated with predicting lung cancer through image classification include the need for large and diverse datasets for training accurate models, the potential for false positives or false negatives in the predictions, and the need for continuous improvement and validation of the classification algorithms.
In conclusion, image classification has shown promising results in predicting lung cancer. By analyzing medical images such as CT scans, machine learning algorithms can accurately identify patterns and abnormalities associated with lung cancer. This technology has the potential to assist healthcare professionals in early detection and diagnosis, leading to improved patient outcomes and survival rates. However, further research and validation are needed to ensure the reliability and effectiveness of these predictive models in real-world clinical settings.