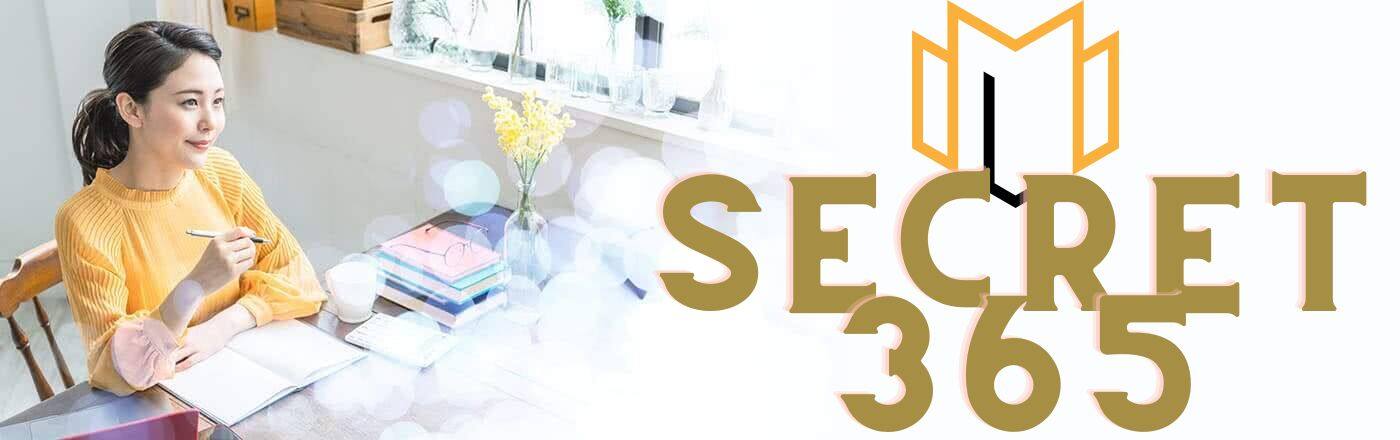
Unleashing the Power of Data Science: A Holistic Approach to Diverse Applications
Data science has emerged as a powerful field that utilizes various techniques and tools to extract meaningful insights from vast amounts of data. Its applications span across numerous industries, making it a crucial component in decision-making processes. This article aims to provide a comprehensive perspective on the diverse applications of data science. By exploring its various domains, such as healthcare, finance, marketing, and more, we can gain a deeper understanding of how data science is revolutionizing these industries and driving innovation.
Data science has emerged as a powerful tool in various industries, and one area where it has made significant contributions is healthcare. The application of data science in healthcare has revolutionized the way patient outcomes are improved and operational efficiency is enhanced. In this article, we will explore the diverse applications of data science in healthcare and how it is transforming the industry.
One of the key areas where data science is making a difference in healthcare is in improving patient outcomes. By analyzing large volumes of patient data, data scientists can identify patterns and trends that can help in early detection and prevention of diseases. For example, by analyzing electronic health records, data scientists can identify patients who are at a higher risk of developing certain conditions and provide targeted interventions to prevent the onset of the disease. This not only improves patient outcomes but also reduces healthcare costs by avoiding expensive treatments.
Data science is also playing a crucial role in personalized medicine. By analyzing genetic data, data scientists can identify genetic markers that are associated with certain diseases or drug responses. This information can be used to develop personalized treatment plans for patients, ensuring that they receive the most effective and safe treatments. This approach has the potential to revolutionize the field of medicine by moving away from a one-size-fits-all approach to a more personalized and targeted approach.
Another area where data science is making a significant impact is in operational efficiency. Healthcare organizations generate massive amounts of data on a daily basis, ranging from patient records to financial transactions. By applying data science techniques, organizations can analyze this data to identify inefficiencies and bottlenecks in their operations. For example, data scientists can analyze patient flow data to identify areas where wait times are longer than average and implement strategies to reduce these wait times. This not only improves patient satisfaction but also optimizes resource allocation, leading to cost savings for the organization.
Data science is also being used to improve the accuracy and efficiency of medical diagnoses. By analyzing medical imaging data, data scientists can develop algorithms that can detect abnormalities and assist radiologists in making accurate diagnoses. This not only reduces the chances of misdiagnosis but also speeds up the diagnostic process, allowing patients to receive timely treatment. Additionally, data science techniques can be used to analyze large volumes of medical literature and clinical trial data to identify new treatment options and improve clinical decision-making.
In conclusion, data science is playing a crucial role in improving patient outcomes and operational efficiency in healthcare. By analyzing large volumes of data, data scientists can identify patterns and trends that can help in early detection and prevention of diseases. They can also develop personalized treatment plans based on genetic data, improving the effectiveness and safety of treatments. Furthermore, data science techniques can be used to optimize operational efficiency, reduce wait times, and improve the accuracy and efficiency of medical diagnoses. As the field of data science continues to evolve, its applications in healthcare are likely to expand, leading to further advancements in patient care and operational efficiency.
Data Science in Finance: Enhancing Risk Management and Predictive Analytics
Data science has revolutionized various industries, and one area where its impact has been particularly significant is finance. With the vast amount of data generated in the financial sector, data science techniques have become invaluable in enhancing risk management and predictive analytics. In this article, we will explore the diverse applications of data science in finance, providing a comprehensive perspective on its role in this field.
Risk management is a critical aspect of the financial industry, and data science has proven to be a game-changer in this regard. By analyzing historical data and identifying patterns, data scientists can develop sophisticated models that assess and predict potential risks. These models enable financial institutions to make informed decisions and take proactive measures to mitigate risks. Whether it is credit risk, market risk, or operational risk, data science provides the tools to identify and manage these risks effectively.
Predictive analytics is another area where data science has made significant strides in finance. By leveraging machine learning algorithms, data scientists can analyze vast amounts of financial data to make accurate predictions about future trends and outcomes. This enables financial institutions to make informed investment decisions, optimize portfolio management, and identify potential market opportunities. Predictive analytics also plays a crucial role in fraud detection, helping to identify suspicious transactions and prevent financial crimes.
One specific application of data science in finance is algorithmic trading. With the advent of high-frequency trading and the increasing complexity of financial markets, data science has become indispensable in developing trading strategies. By analyzing historical market data and identifying patterns, data scientists can create algorithms that automatically execute trades based on predefined rules. This not only improves trading efficiency but also reduces human error and minimizes the impact of emotional decision-making.
Data science has also transformed the field of credit scoring. Traditionally, creditworthiness was assessed based on limited information, such as credit history and income. However, data science techniques have enabled financial institutions to incorporate a wide range of data sources, including social media activity and online behavior, to assess creditworthiness more accurately. This has opened up opportunities for individuals with limited credit history to access financial services and has improved the overall accuracy of credit scoring models.
Furthermore, data science has played a crucial role in the development of robo-advisors. These automated investment platforms leverage data science algorithms to provide personalized investment advice to individual investors. By analyzing an investor's financial goals, risk tolerance, and market conditions, robo-advisors can recommend suitable investment strategies. This democratizes access to financial advice and empowers individuals to make informed investment decisions without the need for traditional financial advisors.
In conclusion, data science has revolutionized the finance industry by enhancing risk management and predictive analytics. From developing sophisticated risk models to enabling algorithmic trading and improving credit scoring, data science techniques have become indispensable in the financial sector. The applications of data science in finance are diverse and continue to evolve, providing financial institutions with valuable insights and empowering individuals to make informed financial decisions. As technology advances and data availability increases, the role of data science in finance is only expected to grow, further transforming the industry and driving innovation.
Data science has become an integral part of various industries, and marketing is no exception. In today's digital age, businesses are constantly seeking ways to gain a competitive edge and maximize their marketing efforts. This is where data science comes into play, as it allows marketers to leverage customer insights for targeted campaigns.
One of the key benefits of data science in marketing is the ability to analyze vast amounts of customer data. With the help of advanced analytics tools, marketers can collect and analyze data from various sources, such as social media, website traffic, and customer surveys. This data provides valuable insights into customer behavior, preferences, and purchasing patterns.
By understanding customer behavior, marketers can create targeted campaigns that resonate with their target audience. For example, data science can help identify the most effective channels to reach customers, whether it's through social media, email marketing, or traditional advertising. This allows marketers to allocate their resources more efficiently and increase the chances of reaching their target audience.
Furthermore, data science enables marketers to personalize their marketing efforts. By analyzing customer data, marketers can segment their audience into different groups based on demographics, interests, or purchasing behavior. This segmentation allows marketers to tailor their messages and offers to specific customer segments, increasing the likelihood of engagement and conversion.
Another application of data science in marketing is predictive modeling. By analyzing historical data, marketers can build models that predict future customer behavior. For example, predictive modeling can help identify customers who are likely to churn or those who are most likely to make a purchase. This information allows marketers to proactively target these customers with personalized offers or retention strategies.
Moreover, data science can also help marketers optimize their marketing campaigns. By continuously monitoring and analyzing campaign performance, marketers can identify areas of improvement and make data-driven decisions. For instance, data science can help determine the optimal timing, frequency, and content of marketing messages. This ensures that marketing efforts are not only targeted but also efficient and cost-effective.
In addition to customer insights, data science can also be used to analyze competitor data. By monitoring competitor activities and analyzing their marketing strategies, businesses can gain a competitive advantage. For example, data science can help identify gaps in the market or uncover new opportunities for product development or marketing campaigns.
However, it is important to note that data science in marketing is not without its challenges. One of the main challenges is data privacy and security. With the increasing amount of customer data being collected, businesses must ensure that they comply with data protection regulations and safeguard customer information.
In conclusion, data science has revolutionized the way marketers approach their campaigns. By leveraging customer insights, personalizing marketing efforts, and optimizing campaigns, businesses can gain a competitive edge in today's digital landscape. However, it is crucial for businesses to prioritize data privacy and security to maintain customer trust. With the continuous advancements in data science, the future of marketing looks promising, with even more opportunities for targeted and effective campaigns.
1. What are some examples of diverse applications of data science?
Some examples of diverse applications of data science include predictive analytics in healthcare, fraud detection in finance, recommendation systems in e-commerce, sentiment analysis in social media, and optimization algorithms in transportation.
2. How does data science contribute to decision-making in various industries?
Data science contributes to decision-making in various industries by analyzing large volumes of data to identify patterns, trends, and insights. This information helps businesses make informed decisions, optimize processes, improve customer experiences, and drive innovation.
3. What are the challenges in implementing data science in different domains?
Some challenges in implementing data science in different domains include data quality and availability, privacy and ethical concerns, talent shortage, integration with existing systems, and the need for continuous learning and adaptation as technologies evolve.
In conclusion, data science has emerged as a powerful tool with diverse applications across various industries. From healthcare and finance to marketing and transportation, data science is being utilized to extract valuable insights, make informed decisions, and drive innovation. The comprehensive perspective of data science allows organizations to leverage their data assets, optimize processes, and gain a competitive edge in the market. As technology continues to advance, the potential for data science to revolutionize industries and improve the quality of life for individuals is immense.