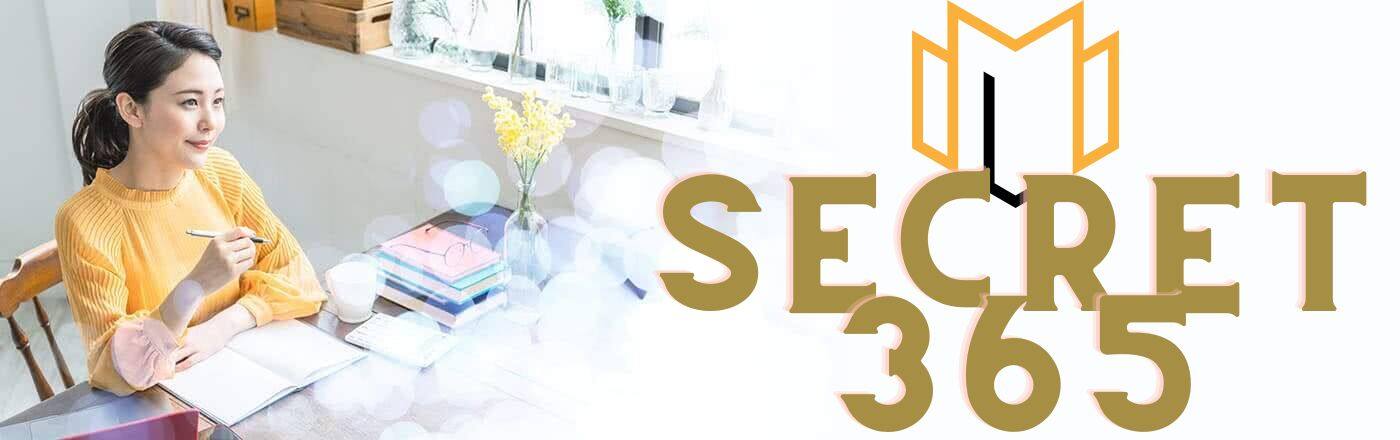
Essential statistical concepts for aspiring data analyst freshers.
Aspiring data analyst freshers need to have a strong foundation in statistical concepts in order to effectively analyze and interpret data. These concepts provide the necessary tools and techniques to make sense of complex datasets and draw meaningful insights. In this article, we will explore some of the must-have statistical concepts that aspiring data analysts should be familiar with in order to excel in their field.
Data analysis is a crucial skill in today's data-driven world, and aspiring data analysts need to have a solid understanding of statistical concepts to excel in their field. In this article, we will introduce some must-have statistical concepts for aspiring data analyst freshers, focusing specifically on descriptive statistics.
Descriptive statistics is the branch of statistics that deals with summarizing and describing the main features of a dataset. It provides a way to organize, summarize, and present data in a meaningful and understandable manner. As a data analyst, you will often be working with large amounts of data, and descriptive statistics will help you make sense of it all.
One of the fundamental concepts in descriptive statistics is measures of central tendency. These measures provide information about the center or average of a dataset. The most commonly used measures of central tendency are the mean, median, and mode. The mean is calculated by summing up all the values in a dataset and dividing by the number of values. The median is the middle value in a dataset when it is arranged in ascending or descending order. The mode is the value that appears most frequently in a dataset. These measures give you a sense of where the data is centered and can help you understand the typical value in a dataset.
Another important concept in descriptive statistics is measures of dispersion. These measures provide information about the spread or variability of a dataset. The range is the simplest measure of dispersion and is calculated by subtracting the minimum value from the maximum value in a dataset. The standard deviation is a more commonly used measure of dispersion and provides a measure of how much the values in a dataset deviate from the mean. A smaller standard deviation indicates that the values are closer to the mean, while a larger standard deviation indicates greater variability. Understanding measures of dispersion is crucial for understanding the spread of data and identifying outliers.
In addition to measures of central tendency and dispersion, data analysts also need to be familiar with graphical representations of data. Graphs and charts provide a visual way to present and analyze data. Some commonly used graphs in descriptive statistics include histograms, bar charts, and scatter plots. Histograms are used to display the distribution of a continuous variable, while bar charts are used to display the distribution of a categorical variable. Scatter plots are used to show the relationship between two continuous variables. These graphical representations can help you identify patterns, trends, and outliers in your data.
Lastly, data analysts need to be aware of the concept of sampling. In many cases, it is not feasible or practical to collect data from an entire population. Instead, data analysts often work with a sample of the population and use statistical techniques to make inferences about the entire population. Understanding sampling methods and the principles of sampling is crucial for ensuring that the sample is representative of the population and that the results can be generalized.
In conclusion, aspiring data analyst freshers need to have a solid understanding of descriptive statistics to excel in their field. Measures of central tendency and dispersion, graphical representations of data, and the concept of sampling are all essential concepts in descriptive statistics. By mastering these concepts, data analysts will be able to effectively analyze and interpret data, providing valuable insights to their organizations.
Understanding Probability and its Applications in Data Analysis
Probability is a fundamental concept in data analysis that plays a crucial role in making informed decisions based on data. Aspiring data analyst freshers must have a solid understanding of probability and its applications to excel in their field. In this article, we will explore some must-have statistical concepts related to probability that every aspiring data analyst should be familiar with.
To begin with, let's define probability. Probability is a measure of the likelihood that a particular event will occur. It is expressed as a number between 0 and 1, where 0 represents impossibility and 1 represents certainty. Understanding probability allows data analysts to quantify uncertainty and make predictions based on available data.
One important concept related to probability is the concept of independent and dependent events. Independent events are those in which the occurrence of one event does not affect the occurrence of another event. For example, flipping a coin twice, where the outcome of the first flip does not influence the outcome of the second flip. On the other hand, dependent events are those in which the occurrence of one event affects the occurrence of another event. For instance, drawing cards from a deck without replacement, where the probability of drawing a certain card changes after each draw.
Another crucial concept in probability is conditional probability. Conditional probability is the probability of an event occurring given that another event has already occurred. It is denoted as P(A|B), where A and B are events. Understanding conditional probability is essential for data analysts as it allows them to analyze data based on specific conditions or constraints.
Bayes' theorem is a powerful tool that is derived from conditional probability. It allows data analysts to update their beliefs or probabilities based on new evidence. Bayes' theorem is particularly useful in situations where prior knowledge or assumptions need to be updated with new data.
In addition to conditional probability, data analysts should also be familiar with the concept of expected value. Expected value is the average value of a random variable over a large number of trials. It is calculated by multiplying each possible outcome by its probability and summing them up. Expected value helps data analysts make decisions by considering the potential outcomes and their associated probabilities.
Furthermore, data analysts should understand the concept of sampling distributions. A sampling distribution is the distribution of a statistic obtained from multiple samples of the same size from a population. It allows data analysts to make inferences about the population based on the sample data. Understanding sampling distributions is crucial for drawing valid conclusions from data and making accurate predictions.
Lastly, data analysts should be familiar with the concept of hypothesis testing. Hypothesis testing is a statistical method used to make inferences about a population based on sample data. It involves formulating a null hypothesis and an alternative hypothesis, collecting data, and using statistical tests to determine the likelihood of the observed data given the null hypothesis. Hypothesis testing helps data analysts make decisions and draw conclusions based on the available evidence.
In conclusion, understanding probability and its applications is essential for aspiring data analyst freshers. Concepts such as independent and dependent events, conditional probability, Bayes' theorem, expected value, sampling distributions, and hypothesis testing are crucial for making informed decisions based on data. By mastering these statistical concepts, aspiring data analysts can enhance their analytical skills and excel in their field.
Statistical concepts are the backbone of data analysis, and aspiring data analyst freshers must have a solid understanding of these concepts to excel in their field. One of the most important statistical concepts to grasp is hypothesis testing, which allows analysts to make inferences about a population based on a sample.
Hypothesis testing involves formulating a null hypothesis and an alternative hypothesis. The null hypothesis represents the status quo or the belief that there is no significant difference or relationship between variables. The alternative hypothesis, on the other hand, suggests that there is a significant difference or relationship.
To test these hypotheses, analysts collect data and perform statistical tests. One commonly used test is the t-test, which compares the means of two groups to determine if they are significantly different. Another popular test is the chi-square test, which examines the association between categorical variables.
In addition to hypothesis testing, statistical inference is another crucial concept in data analysis. Statistical inference involves drawing conclusions about a population based on a sample. It allows analysts to make predictions and generalizations beyond the data they have collected.
One common method of statistical inference is confidence intervals. A confidence interval provides a range of values within which the true population parameter is likely to fall. For example, if a 95% confidence interval for the mean height of a population is 160-170 cm, it means that we can be 95% confident that the true mean height falls within this range.
Another important concept in statistical inference is p-values. A p-value measures the strength of evidence against the null hypothesis. It represents the probability of obtaining the observed data, or more extreme data, if the null hypothesis is true. A p-value less than a predetermined significance level (usually 0.05) indicates that the observed data is unlikely to occur by chance alone, leading to the rejection of the null hypothesis.
Understanding these statistical concepts is essential for aspiring data analyst freshers because they form the basis for making informed decisions and drawing meaningful insights from data. By applying hypothesis testing and statistical inference, analysts can confidently analyze data and provide valuable insights to businesses and organizations.
Moreover, these concepts also help analysts avoid common pitfalls in data analysis, such as drawing incorrect conclusions or making false claims based on insufficient evidence. By following a rigorous statistical approach, analysts can ensure the accuracy and reliability of their findings.
To further enhance their statistical skills, aspiring data analyst freshers should also familiarize themselves with other related concepts, such as statistical power, effect size, and sample size determination. These concepts provide additional tools for conducting robust and meaningful data analysis.
In conclusion, exploring hypothesis testing and statistical inference is crucial for aspiring data analyst freshers. These concepts enable analysts to make informed decisions, draw meaningful insights, and avoid common pitfalls in data analysis. By mastering these statistical concepts, freshers can lay a strong foundation for a successful career in data analysis.
1. What are some must-have statistical concepts for aspiring data analyst freshers?
- Descriptive statistics
- Inferential statistics
- Probability theory
- Hypothesis testing
- Regression analysis
2. Why are these statistical concepts important for aspiring data analyst freshers?
- Descriptive statistics helps in summarizing and interpreting data.
- Inferential statistics allows making predictions and drawing conclusions from a sample to a larger population.
- Probability theory helps in understanding the likelihood of events occurring.
- Hypothesis testing helps in making decisions based on data analysis.
- Regression analysis helps in understanding the relationship between variables.
3. How can aspiring data analyst freshers learn and master these statistical concepts?
- Take online courses or enroll in formal education programs that cover statistics.
- Practice applying statistical concepts to real-world data sets.
- Read books and research papers on statistics and data analysis.
- Seek mentorship or guidance from experienced data analysts.
- Participate in data analysis projects or internships to gain hands-on experience.
In conclusion, aspiring data analyst freshers should have a strong understanding of key statistical concepts. These concepts include probability, hypothesis testing, regression analysis, and data visualization. By mastering these concepts, data analysts can effectively analyze and interpret data, make informed decisions, and communicate findings to stakeholders.