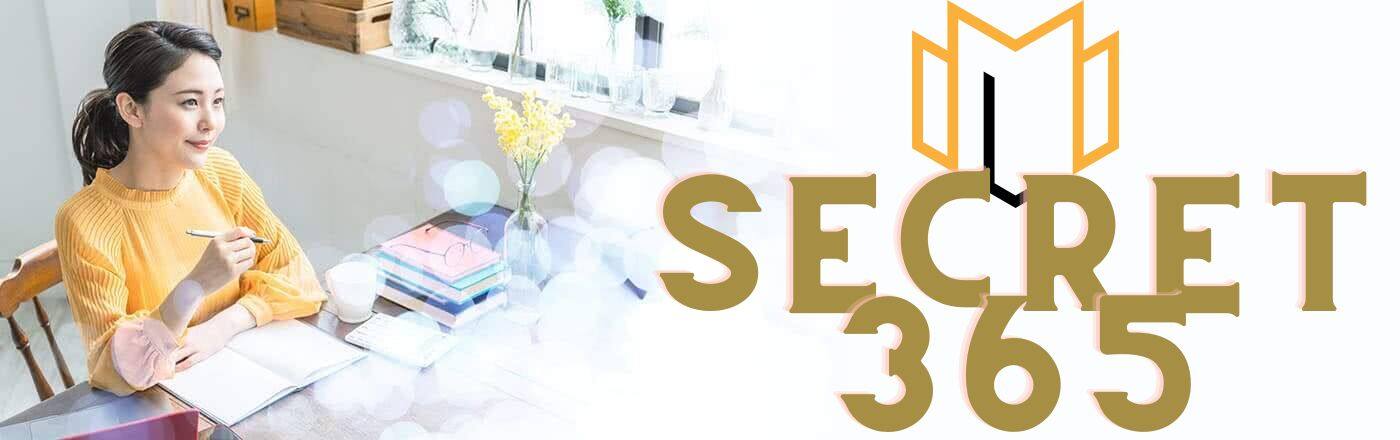
Unleashing the Power of Deep Learning: Transforming Industries
Deep learning, a subset of artificial intelligence, has revolutionized various industries by enabling machines to learn and make decisions like humans. This technology has brought about game-changing applications that are reshaping industries across the globe. From healthcare and finance to transportation and manufacturing, deep learning is being leveraged to solve complex problems, improve efficiency, and drive innovation. In this article, we will explore some of the most impactful deep learning applications that are shaping industries today.
Deep learning, a subset of artificial intelligence (AI), has emerged as a game-changer in various industries. One sector that has witnessed a significant transformation due to deep learning is healthcare. With its ability to analyze vast amounts of data and identify patterns, deep learning has revolutionized the way healthcare professionals diagnose diseases, develop treatment plans, and improve patient outcomes.
One of the key applications of deep learning in healthcare is medical imaging. Traditional methods of analyzing medical images, such as X-rays and MRIs, often require human interpretation, which can be time-consuming and prone to errors. Deep learning algorithms, on the other hand, can quickly and accurately analyze these images, helping doctors detect abnormalities and make more accurate diagnoses. This not only saves time but also improves the accuracy of diagnoses, leading to better treatment outcomes for patients.
Another area where deep learning is making a significant impact is in drug discovery and development. Developing new drugs is a complex and expensive process that often takes years. Deep learning algorithms can analyze vast amounts of data, including genetic information, clinical trial results, and scientific literature, to identify potential drug candidates. This accelerates the drug discovery process, allowing researchers to focus their efforts on the most promising candidates, ultimately leading to the development of more effective and targeted therapies.
Deep learning is also being used to personalize treatment plans for patients. By analyzing a patient's medical history, genetic information, and lifestyle factors, deep learning algorithms can predict how an individual is likely to respond to a particular treatment. This enables healthcare professionals to tailor treatment plans to each patient's unique needs, improving the chances of successful outcomes and reducing the risk of adverse reactions.
Furthermore, deep learning is playing a crucial role in predicting and preventing diseases. By analyzing large datasets, including electronic health records and genetic information, deep learning algorithms can identify patterns and risk factors associated with various diseases. This allows healthcare providers to proactively intervene and implement preventive measures, potentially saving lives and reducing healthcare costs.
In addition to these applications, deep learning is also being used to improve the efficiency of healthcare operations. By analyzing data from electronic health records, deep learning algorithms can identify patterns and trends that can help hospitals and healthcare systems optimize resource allocation, streamline workflows, and improve patient flow. This not only improves the overall efficiency of healthcare delivery but also enhances the patient experience.
Despite the numerous benefits of deep learning in healthcare, there are also challenges that need to be addressed. One of the main challenges is the need for large and diverse datasets to train deep learning algorithms effectively. Additionally, ensuring the privacy and security of patient data is of utmost importance to maintain trust and compliance with regulations.
In conclusion, deep learning is revolutionizing healthcare by enabling faster and more accurate diagnoses, accelerating drug discovery, personalizing treatment plans, predicting and preventing diseases, and improving the efficiency of healthcare operations. While there are challenges to overcome, the potential of deep learning to transform healthcare is immense. As technology continues to advance, we can expect even more innovative applications of deep learning that will further shape the future of healthcare.
Deep learning, a subset of artificial intelligence (AI), has revolutionized various industries by enabling machines to learn and make decisions without explicit programming. One sector that has greatly benefited from deep learning applications is autonomous vehicles and transportation. With the ability to process vast amounts of data and learn from it, deep learning algorithms have paved the way for safer and more efficient transportation systems.
One of the key applications of deep learning in autonomous vehicles is object detection and recognition. Traditional computer vision techniques often struggle to accurately identify objects in complex and dynamic environments. However, deep learning algorithms excel at this task by analyzing large datasets and learning patterns and features that are crucial for object recognition. By leveraging deep learning, autonomous vehicles can accurately detect and classify objects such as pedestrians, cyclists, and other vehicles, enabling them to make informed decisions and navigate safely.
Another significant application of deep learning in transportation is natural language processing (NLP). NLP allows machines to understand and interpret human language, which is essential for developing intelligent voice assistants and communication systems in autonomous vehicles. Deep learning models, such as recurrent neural networks (RNNs) and transformers, have been trained on vast amounts of text data, enabling them to understand and respond to human commands and queries. This technology not only enhances the user experience but also improves the overall safety of autonomous vehicles by reducing driver distraction.
Deep learning also plays a crucial role in predictive maintenance for transportation systems. By analyzing sensor data from various components of vehicles, deep learning algorithms can detect patterns and anomalies that indicate potential failures or maintenance needs. This proactive approach to maintenance helps prevent costly breakdowns and ensures the reliability and longevity of transportation infrastructure. Moreover, deep learning models can continuously learn from new data, allowing them to improve their predictions over time and adapt to changing conditions.
Traffic management is another area where deep learning is making a significant impact. Deep learning algorithms can analyze real-time traffic data, including vehicle speeds, congestion levels, and accident reports, to predict traffic patterns and optimize traffic flow. By leveraging this technology, transportation authorities can make informed decisions regarding traffic signal timings, lane management, and route planning, leading to reduced congestion, shorter travel times, and improved overall efficiency of transportation networks.
Furthermore, deep learning is being used to enhance the safety and security of autonomous vehicles. Advanced driver assistance systems (ADAS) powered by deep learning algorithms can monitor the vehicle's surroundings, detect potential hazards, and provide timely warnings or take corrective actions. These systems can also analyze driver behavior and detect signs of fatigue or distraction, alerting the driver or even taking control of the vehicle if necessary. By integrating deep learning into ADAS, the industry is moving closer to fully autonomous vehicles that can navigate and respond to complex situations with minimal human intervention.
In conclusion, deep learning applications have brought about a paradigm shift in the autonomous vehicles and transportation industry. From object detection and recognition to natural language processing, predictive maintenance, traffic management, and safety enhancements, deep learning algorithms are transforming the way we travel and commute. As technology continues to advance, we can expect even more game-changing applications of deep learning that will shape the future of transportation, making it safer, more efficient, and more sustainable.
Deep learning, a subset of artificial intelligence (AI), has been making waves across various industries, revolutionizing the way businesses operate. One sector that has particularly benefited from deep learning is retail and e-commerce. With its ability to analyze vast amounts of data and extract meaningful insights, deep learning has transformed the customer experience in this industry.
One of the key areas where deep learning has had a significant impact is in personalized recommendations. Retailers and e-commerce platforms are constantly striving to provide customers with tailored product suggestions that match their preferences. Deep learning algorithms have made this possible by analyzing customer data, such as purchase history, browsing behavior, and demographic information, to generate accurate recommendations. By understanding individual preferences, retailers can now offer a more personalized shopping experience, increasing customer satisfaction and loyalty.
Another way deep learning has transformed the customer experience in retail and e-commerce is through image recognition. With the rise of social media and online shopping, customers often come across products they like but may not know the brand or name. Deep learning algorithms can analyze images and identify specific products, enabling customers to easily find and purchase them. This technology has not only made it easier for customers to discover new products but has also opened up new marketing opportunities for retailers.
Furthermore, deep learning has played a crucial role in improving inventory management and supply chain optimization. Retailers often struggle with predicting demand accurately, leading to either excess inventory or stockouts. Deep learning algorithms can analyze historical sales data, market trends, and external factors to forecast demand more accurately. This enables retailers to optimize their inventory levels, reducing costs and improving overall efficiency. Additionally, deep learning can help identify potential bottlenecks in the supply chain, allowing retailers to address them proactively and ensure timely delivery to customers.
In addition to these applications, deep learning has also been instrumental in enhancing fraud detection and prevention in retail and e-commerce. With the increasing prevalence of online transactions, fraudsters have become more sophisticated in their methods. Deep learning algorithms can analyze vast amounts of data, including transaction history, customer behavior, and external factors, to identify patterns indicative of fraudulent activity. By detecting and preventing fraud in real-time, retailers can protect their customers and maintain trust in their platforms.
The impact of deep learning in retail and e-commerce is not limited to customer-facing applications. It has also transformed back-end operations, such as inventory management, pricing optimization, and customer service. By automating repetitive tasks and providing data-driven insights, deep learning has enabled retailers to streamline their operations and make more informed decisions.
In conclusion, deep learning has revolutionized the customer experience in retail and e-commerce. From personalized recommendations to image recognition, inventory management to fraud detection, deep learning applications have transformed the way businesses operate in this industry. By leveraging the power of AI, retailers can now provide a more personalized and seamless shopping experience, ultimately driving customer satisfaction and loyalty. As deep learning continues to evolve, we can expect even more game-changing applications that will shape the future of retail and e-commerce.
1. What are some examples of deep learning applications shaping industries?
Some examples include autonomous vehicles, medical diagnosis, and natural language processing.
2. How is deep learning being used in autonomous vehicles?
Deep learning is used in autonomous vehicles for tasks such as object detection, lane detection, and decision-making algorithms.
3. What role does deep learning play in medical diagnosis?
Deep learning is used in medical diagnosis for tasks such as image analysis, disease classification, and predicting patient outcomes.
In conclusion, deep learning applications have emerged as game-changers in various industries. These applications have revolutionized fields such as healthcare, finance, transportation, and manufacturing, among others. By leveraging the power of neural networks and large datasets, deep learning has enabled significant advancements in areas like medical diagnosis, fraud detection, autonomous vehicles, and predictive maintenance. The potential of deep learning to transform industries is immense, and its continued exploration and development hold promise for further innovation and progress.